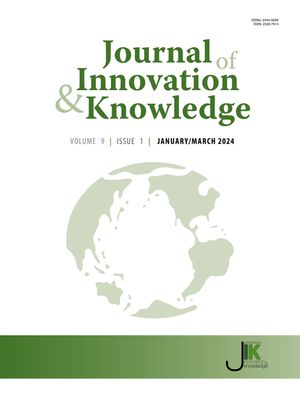
Edited by: Abbas Mardari
More infoIn recent years, both information technology (IT) labor demand and IT job characteristics have been significantly influenced by the advent of Industry 4.0 (I4.0) and the digital age. The higher education sector is expected to upgrade itself with such progress and train graduates capable of meeting the new industry requirements. However, due to the present demand gap, universities are seeking to identify the skills new digital workers are expected to have. As we live in the digital age, the literature has failed to address the questions of the consequences for individual workers adequately. Considerable scientific efforts should be made to design certain curricula and training programs that could nurture digital competencies (DC). There is a need to construct an inclusive framework of the term. In addition, different options exist for studying the DC of the workforce in relation to human resources management (HRM). The current paper attempts to fill the gap by developing an integrated decision-making framework. The paper aims to introduce an innovative discrimination measure and discuss its elegant properties. A Measurement of Alternatives and Ranking according to the COmpromise Solution (MARCOS) framework for evaluating the MCDM problem on a fuzzy set has been developed based on it. On the other hand, the new methodology of the PF-entropy-RS weight technique is used to compute the criteria weights or significance degrees of criteria. Then, the MARCOS method is a new elegant approach to handle the MCDM problems. Thus, in this study, we have developed a new approach to the multi-criteria decision-making (MCDM) method using the PF-entropy-RS and PF-MARCOS methods and further implemented for the evaluation of the competencies of the future workforce for digital technologies implementation in higher education. It helps to calculate the objective/subjective weights of criteria to evaluate the competencies of the future workforce for digital technologies implementation in higher education; then, the approach is used to assess the preferences of higher education institutes over different competencies of the future workforce for digital technologies implementation in higher education. An empirical case study is carried out in this paper for the evaluation of the most important competencies of the future workforce for digital technologies implementation in higher education. In addition, to evaluate the performance quality of the proposed framework, this study also involves some comparison and sensitivity analyses. This study found that data and information processing has emerged as the most important competencies of the future workforce for digital technologies implementation in higher education. The ability to interact with modern interfaces is the second most important competency of the future workforce for digital technologies implementation in higher education. In conclusion, the workforce responsible for implementing digital technologies in higher education will significantly impact students’ learning experiences. As technological advancements continue, higher education institutions will require skilled professionals with diverse digital expertise to manage and integrate these technologies successfully.
In recent decades, the term ‘competency’ has appeared in many situations and different domains. This term has not only been widely discussed in the work setting and educational subjects, but also it has been a great concern in individuals’ personal and societal lives (Barth et al., 2007). Tremendous societal changes, the technology development, and the globalization phenomenon have caused novel challenges, e.g., the growth of societal diversity, growth of individualization, expansion of cultural and economic uniformity, the generation of massive information, and the increase of complexity and uncertainty (Rychen & Salganik, 2001). According to Campion et al. (2011), competence refers to the “collections of knowledge, skills, abilities and other characteristics that are needed for effective performance in the jobs in question” (Campion et al., 2011; Shippmann et al., 2000; Rodriguez et al., 2002; Schlegel & Kraus, 2023). The Fourth Industrial Revolution (Industry 4.0) requires company staff to concentrate on creative, innovative, and communicative activities. In this context, workers are expected to be capable of performing more complicated and indirect tasks, for example, working with collaborating robots and machines (Levy & Murnane, 2013). In addition, they must deal with information produced in real-time and higher quantities of data and be able to communicate with machines (Gehrke et al., 2015). Though, machines often perform repetitive tasks (Lanza et al., 2015). The challenge is that there is still no agreement on the definition of important competencies, and no strategy has yet been established clarifying the way the approach of competence acquisition could be incorporated into higher education (Barth et al., 2007). Generally, competencies might be characterized as dispositions to self-organization, which comprise a variety of psycho-social components. Individuals could slowly obtain these competencies in various phases, which could finally be reflected in performing required activities successfully. Moreover, the term ‘key competency’ is highly important since it characterizes a qualitative extension mentioning the special importance of particular competencies (Busulwa et al., 2022).
Key competencies could be thought of as important to all individuals in their various spheres of life (Alm et al., 2022; Rychen & Salganik, 2003; Yang, 2023). Remember that key competencies are not a substitute for domain-specific competencies essential for acting successfully in particular conditions and contexts. Key competencies bear a different and wider focus; they pool various competency classes and are positioned transversely to them. These types of competencies are consisted of various domain-specific capabilities and identify the most significant competency areas. To deeply understand the key competency concept, there is a need for a comprehensive definition of the term and information regarding the key competencies of certain significance. It is worth mentioning that any attempt to compile an all-inclusive scheme regarding all possible and essential key competencies will fail immediately because such a list must terminate unpredictably (Weinert, 2001). Thus, employees are required to be well-engaged in lifetime learning to achieve new competencies that are necessary for being well-adapted to the rapidly-changing work environment (Carnevale & Smith, 2013; Cascio & Montealegre, 2016; Oberländer et al., 2020). In fact, the literature shows a break between the existing and the required digital competencies (DC) of the workforce (Ancarani & Di Mauro, 2018; Janssen et al., 2013). As reported by Arnold et al. (2016), more than 60 % of the interviewed workers pointed out that they were continually required to raise their competencies at the worksite due to the rapid emergence of technical innovations. Although employers and policymakers have shown to be aware of this issue, the literature still lacks scientific research concentrating on workers’ DC (Murawski & Bick, 2017).
The ongoing digitalization has hugely affected people's everyday social lives; beyond that, innovative technologies have also tremendously impacted the way people work (Murawski & Bick, 2017; Zaphiris & Ioannou, 2015). Both hardware and software employed in specialized settings are continuously improved to offer higher efficiency and make jobs easier. This condition has led to the growth of the use of digital ICT. The majority of worksites need individuals to hold at least basic DCs (Gallardo-Echenique et al., 2015). In the guidelines of the European Parliament and the Council, competence was described as a combination of skills, knowledge, and attitudes, and DC was defined as “the confident and critical use of Information Society Technology for work, leisure and communication” (Søby, 2013).
In the path towards developing key competencies, higher education must reorient the learning processes as a core task. To this end, there is a need for a “new learning culture” (Barth et al., 2007) that can move away from a learning-based system into an “enabling-oriented, based on self-organization and centered on competence” (Erpenbeck & Rosenstiel, 2003). In this sense, Arnold and Lermen (2005) highlighted the need for establishing an “enabling didactic”, aiming to provide professional training and promote personality development and make individuals capable of coping with complicated situations, acting upon reflection, and making effective decisions accordingly. In addition, the concept of enabling didactic seeks to make individuals capable of taking on responsibility, considering ethical standards when acting, and judging the consequences.
Higher education is experiencing a rapid evolution in its role in modern society; owing to globalization and its related issues, a desired student is defined as a student who can succeed within a varied and interrelated economy (Di Pietro et al., 2015; Huang, 2017). Today, the principal objective of graduate programs is preparing students for a globalized world (Di Pietro, 2015; Islam & Stamp, 2020; Zevallos, 2012). As a result, to stay competitive in the present world, higher education needs to support students to learn many relevant competencies (Zevallos, 2012) successfully.
Industry 4.0 (I4.0) (Galaske et al., 2018; Kagermann et al., 2013; Kargas et al., 2022; Lista Rossetti et al., 2023) designates the future production systems that are impacted by digital manufacturing technologies. I4.0 aims to provide consumers with individualized items in small consignments (instead of the conventional mass production system) by enabling an adaptive production procedure and optimizing the value chains and value-added networks (Galaske et al., 2018). Although automation is implemented increasingly in the industry, the human factor still has a considerable role in I4.0, particularly in making production processes highly flexible.
At the present time, the higher education sector faces a paradigm shift (Gosselin et al., 2013, 2016). Several articles have maintained that this sector requires employers to be able to satisfy future workforce demands (Jackson & Meek, 2021; Rotatori et al., 2021). Higher education is now facing a specific challenge to enhance students’ competency, including creativity, collaboration, communication, critical thinking, innovation, problem-solving, and self-management (Ferreras-Garcia et al., 2021; Martha et al., 2021), which are recognized as the “21st-century skills” or 21st-century competencies. The competencies approach implemented worldwide a few years ago in teaching/learning activities originated from the social changes that occurred during the recent decades. Though, that approach terminates with the rapid advent and expansion of globalization. In fact, some instances of this digital revolution include the continuous revitalization of ICT, the exponentially-increasing volume of data, the implementation of various data resources and digital media and their extraordinary utilization (Basilotta-Gómez-Pablos et al., 2022), the call for learning stages that can provide distance teaching/learning and the initiation of emergency remote teaching (ERT) (Trust & Whalen, 2020) accelerated by the COVID-19-induced health emergency.
Nowadays, a key challenge the higher education sector faces is how to properly prepare students to satisfy future demands for the workforce (Bellanca & Brandt, 2010; Craps et al., 2022; Zemsky, 2009). Both policymakers and business practitioners are asking schools and higher education institutions to grow twenty-first-century competencies in their students. Such competencies are considered a basis for both collective and individual success in the workplace; however, many employers have reported considerable shortages of these skills. For that reason, business leaders and educational organizations have emphasized the need for novel education policies aiming to develop wide and transferable knowledge and skills.
Therefore, DC has been highly important in educational contexts; it is one of the most important competencies teachers need to succeed. Most of the approaches/models are concentrated on the pre-university levels, although the academic community is increasing interest in recognizing the DC level of university teachers. This attribute generally refers to the skills, understanding, and outlooks a teacher requires to implement the available technologies effectively. This is the way DC has recently attained high significance in the educational context (Tejada & Pozos, 2018). There are two main reasons for this trend: 1) the technology implementation has gotten into a routine, and 2) the individuals’ professional progress is largely dependent on the efficient implementation of ICT. As per Cabero-Almenara et al. (2020), in future societies, DC is a key competency that people, generally, and teachers, particularly, need to master.
The literature consists of evidence indicating that teachers have modest levels of DC (Guillén-Gámez & Mayorga-Fernández, 2020; Romero-Rodríguez et al., 2019). Such skills include solving emerging problems in the workplace through ICT, working with others through a network, and implementing 2.0 tools for evaluation purposes. In addition, the above list was added by Blayone et al. (2018) with social competence, technical competence, informational competence, as well as epistemological competence; the last item has been reported to be the poorest one. According to Basantes-Andrade et al. (2020), unlike age, gender does not influence the individuals’ level of DC (Cabero-Almenara et al. (2021). Findings have shown that teachers aged between 30 and 49 years and those who have 4–14 years of experience demonstrate higher levels of DC.
Along with the serious change to the challenges, potentials, and requirements of industrial companies, the competencies they need to survive in the future market have changed, too (Hirsch-Kreinsen, 2014). Workforce competencies are key to succeeding in the current extremely innovative and connected industry (Gehrke et al., 2015). This could be achieved by focusing on providing the (future) workforce with the essential competencies (Gehrke et al., 2015). Reviewing the literature related to the industry shows a focus on the content of educational intuitions (Enke et al., 2018). For this concept to be well developed, first, there was a need for the evaluation of the competencies the future workforce needs to have to survive in the digitalization era. This study reviewed the existing literature to determine the competencies the future workforce requires to implement digital technologies in higher education effectively.
To make use of the efficiency and flexibility of PFSs, this study introduced a new discrimination measure with well-designed characteristics. Accordingly, the MARCOS framework was constructed in order to assess the MCDM problem on PFSs. In addition, this study used the novel method of PF-entropy-RS weight to find a technique for the calculation of the weights or significance degrees of the criteria. MARCOS is, in fact, an innovative approach designed to deal with MCDM problems in an effective way. For that reason, the current paper developed a novel approach to MCDM using the PF-entropy-RS and PF-MARCOS methods and further implemented it to evaluate the competencies of the future workforce for digital technologies implementation in higher education. In the following, the main objectives of this study are presented as
- ■
To perform survey research using current literature review and interviews with experts to identify the competencies of the future workforce for digital technologies implementation in higher education.
- ■
To develop an inclusive framework for the identification of the competencies of the future workforce for digital technologies implementation in higher education.
- ■
To propose an integrated decision-making approach on PFSs using the PF-entropy-RS and PF-MARCOS models to rank the institutes and analyze and assess the competencies of the future workforce for digital technologies implementation in higher education.
- ■
The PF-entropy-RS approach is applied to evaluate and rank the competencies of the future workforce for digital technologies implementation in higher education.
- ■
To present the sensitivity and comparison analyses to confirm the robustness of the introduced PF-entropy-RS-MARCOS methodology.
The remainder of this paper is provided in the following way: Section 2 presents the literature review related to the competencies of the future workforce for digital technologies implementation in higher education. Section 3 provides the proposed PF-entropy-RS-MARCOS method and the fundamentals of PFSs. Section 4 presents the results of the study, the case study, the sensitivity investigation, and the comparative study. At last, section 5 discusses the conclusions of the study.
Future workforce competencies for digital technologies in higher educationNotwithstanding the desires of the academic community for the development of a workforce capable of delivering across-sectors skills, the question of ‘how to implement’ has still remained a challenge. A factor that may have contributed to this challenge is the fact that in the past, there was a tendency to strengthen the workforce only through formal professional education programs; although, the workforce needs strategies for continuously supporting and evaluating the competency development, maintenance, and progression a daily basis over time (Barbazza et al., 2015; Levkovich, 2015). Even in cases where the workforce's competency is continuously developed and assessed, there are still several challenges for reinforcing the competency across companies; there is a need for system-wide change with the alignment of operations, planned partnership working, and developing and assessment the competency in all of the care sectors.
According to Nicholson et al. (2013) and Barbazza et al. (2015), it is necessary to provide qualified competency development programs and evaluation processes under widely-accepted standards. The literature consists of several examples of innovations in the development of qualified programs; however, it lacks enough research on how people's competencies can be evaluated effectively. Kiljunen et al. (2019) attempted to identify the challenges arising when employing self-assessment as a competence measure for nurses. They also considered the difference between manager-assessed and self-assessed competency; however, the cross-sector assessment was not considered. Thomson et al. (2018) highlighted several inconsistencies across organizations in terms of how care certificates are used, while the cross-sector assessment was not investigated. Their study included the differences in the ways training is delivered and staff's competencies are evaluated. According to Thomson et al. (2018), inconsistency between organizations undermines the portability and credibility of the Care Certificate. After reviewing the quality improvement tools, Varkey et al. (2007) suggested that audits and feedback could greatly help identify the variations and gaps in competencies. They focused on the implementation of improvement tools to evaluate competencies; however, they failed to clarify the way these are related to the evaluation of the competencies of individuals who work in a cross-organizational context. As suggested by Lockyer (2003), multi-source or 360-degree assessments can effectively assess the individuals’ competencies by considering interpersonal and communication skills, systems-based practices, and professionalism. On the other hand, these assessments were shown to be less appropriate for the evaluation of clinical and practice-based competencies. In the context of Australia, Palermo et al. (2015) studied the dietician graduates’ experiences of competency evaluation, which revealed that the competence evaluation within work-based settings might suffer from subjectivity and inconsistency mainly due to the implementation of different standards across sites. According to Cusack and Smith (2010), competency evaluation could face some challenges where perceived power inequalities exist as a consequence of a complicated workforce environment, healthcare cultures, and professional hierarchies.
There are several blogs in higher education with the objective of developing linguistic, digital, and interpersonal competencies (Neira Piñeiro et al., 2013). According to Esteban, (2017), the utilization of video positively affects the attainment of DC. They also maintained that in cases where videos are utilized as learning objects, the individuals’ informational competencies enhance. In another research (Ruiz Cabezas et al., 2020), it was revealed that teachers were interested in integrating DC into teaching practices and materials and generating a context for professional development.
Even with such discussions, until the early years of the 1990s, the literature has witnessed relatively little attention to the concept of competency. It became more important when (Prahalad & Hamel, 1997) published their influential study on core competencies. After that, it became a dominant concept in Dutch organizations/companies. Remember that the Dutch economy is widely known as the knowledge economy. Flexibility was recognized as the key to balance in the debate on curricula and education (van Zolingen, 1995); numerous scholars and practitioners have indicated that it was neither desirable nor realizable to directly translate the demands of the labor market into educational curricula. The concept of key qualifications was introduced by Mertens (1974) in the 1970s in Germany. This concept is mainly concentrated on the skills, understanding, and outlooks required for individuals to perform flexibly in a certain occupational domain. Then, in the last years of the 1990s, the key qualifications concept gradually made the stage ready for the concept of competencies that was about teachable and learnable attributes indicating the individuals’ aptitudes for performing different human activities, for instance, working, learning, and adapting with changes. Higher education has increasingly appeared as a sector; thereby, the focus was shifted to competencies such as problem-solving, teamwork, analytical capabilities, and creativity. Such competencies were regarded as the characteristics of a graduate who is competent and employable.
The digital age and I4.0 substantially impact both information technology (IT) job characteristics and IT labor demand. The managers in the higher education sector need to cope well with the situation by producing graduates equipped with the quality and preparation so that the industry requirements can be well satisfied. However, given the present demand gap, universities show enthusiasm to first know the skills that IT-related industries require new digital workers to have. Currently, the world is going through the 4th industrial revolution; this age has witnessed an increase in both the volume and worth of knowledge with a high level of accessibility (Baller et al., 2016). The huge changes that have occurred in consumers’ consumption and the quick development of technology have affected the business evolution (Buhalis & Amaranggana, 2013; Labrecque et al., 2013; Ritzer & Jurgenson, 2010) and caused the emergence of many novel business models in different sectors. For instance, Airbnb1, which has newly appeared in the travel and tourism context, employs the concept of sharing accommodations, which can provide income to owners; in addition, the transportation business has recently provided increased choices to customers with the idea of ride-sharing. The business evolution has injected high flexibility into jobs in this context. Such changes have directly impacted the IT workforce's job characteristics (Gretzel et al., 2015; Leahy & Wilson, 2014).
I4.0 designates the future production vision that is under the influence of digitalization. Even with the growing degree of automation, human factors greatly affect the facilitation of a production process of high flexibility. It causes the workforce to have new requirements through novel technologies, organizational forms, and workflows. The workforce managers have to take into consideration novel competencies required in the era of I4.0 and digitalization. The increasingly developing assistance systems require the workforce to be well-trained in properly interacting with and handling these systems.
Such digital transformation also results in an increase in the organizational/technological complexity of manufacturing (Schuh et al., 2014). The changes that occurred to the challenges, potentials, and requirements of industrial firms caused a great change to the competencies of the workforce working in the industry sector (Hirsch-Kreinsen, 2014). In greatly innovative and connected factories, the worker's and employees’ competencies are a critical key to success (Gehrke et al., 2015). There is a need to emphasize training the (future) workforce to achieve the company's potential in the future market (Armstrong & Taylor, 2020). Scholars and practitioners believe that educational intuitions’ focal points and contents have changed significantly (Enke et al., 2018). DC refers to some basic knowledge, capabilities, skills, and other features enabling individuals at work to do their tasks efficiently and successfully. We selected the term ‘digital’ since this is a moderately wide-ranging term that encompasses any type of ICT, digital media, applications, and devices used at work, at the present time, and in the future. However, according to above discussions, in this study, we have identified several competencies of the future workforce that are: C1: IT/ ICT competencies, C2: Innovation capability, C3: Working in interdisciplinary environments, C4: Analytical skills, C5: Implementation of ideas in the shop floor, C6: Conflict solving self-and time management, C7: Social intelligence, C8: Creativity, C9: Exchange of opinions and ideas, C10: Commitment to lifelong learning, C11: Ability to communicate about complex problems, C12: Generic knowledge for production and technologies processes, C13: Mindset for building and maintaining networks of experts, C14: Personal flexibility, C15: Problem solving, C16: Data and information processing, C17: Collaboration skills, C18: Decision making, C19: Teamwork, C20: Value-driven management style, C21: Ability to solve complex concepts for realistic work packages use by appropriate people, C22: Ability to interact modern interfaces, C23: Encouragement taking new routes, C24: Understanding of interrelations technological components and C25: Process understanding.
The proposed PF-entropy-RS-MARCOS methodologyThe characterization of the intuitionistic fuzzy sets (IFSs) theory is based on the degrees of belongingness and non-belongingness with their sum bounded to 1 (Atanassov, 1986). In the decision-making problem, some conditions may appear where the decision experts (DEs) provide a value of 0.8 if an alternative fulfills the criterion and a value of 0.5 if the alternative dissatisfies the criterion. In such conditions, 0.8+0.5>1; as a result, IFS cannot be used to cope with such a situation (Yager, 2014; Rani et al., 2020a). To find a solution to this problem, Yager (2013) initiated the idea of Pythagorean fuzzy sets (PFSs). Therefore, PFSs could be more relied upon compared to IFSs when describing the nature of ambiguity. In recent years, the complex proportional assessment (COPRAS) method was developed by Alipour et al. (2021) under PFSs for the assessment of fuel cell and hydrogen components suppliers. In another study, a new PF-WASPAS method with frank aggregation operators was proposed by Wang and Zhang (2022) for cloud computing product selection. With the use of the PF-CoCoSo approach, Liao et al. (2020) assessed and prioritized the cold chain logistics distribution centers. Mishra et al. (2022) studied a similarity measure-based additive ratio assessment (ARAS) framework for assessing biomass crops from sustainable perspectives.
For the sensible aggregation of prioritizations, the “measurement alternatives and ranking based on the compromise solution (MARCOS)” approach was given by Stević et al. (2020), which comprises as (i) considering “ideal solution (IS)” and “anti-ideal solution (AIS)” as reference points, (ii) setting up the relationship between options and IS/AISs, (iii) expressing the “utility degree (UD)” of each option in association to ISs and AISs. Chakraborty et al. (2020) proposed a hybridized D-MARCOS method for solving the supplier selection of the iron and steel industry. Bakır and Atalık (2021) combined the analytic hierarchy process and MARCOS methods with fuzzy numbers to handle the uncertainty of the e-service evaluation problem. Ali (2022) studied a hybrid score function-based q-rung orthopair fuzzy MARCOS methodology for assessing the solid waste management system. An integrated MARCOS method based on prospect theory and the neutrosophic cubic set has been introduced by Fan et al. (2022) to solve the MCDM problems. Thus far, there is no study regarding a hybrid PF-entropy-rank sum-MARCOS methodology from a Pythagorean fuzzy perspective for the evaluation of the competencies of the future workforce for digital technologies implementation in higher education.
In the subsection, we present some fundamental ideas related to the PFSs.
Definition 1 (Yager, 2013, 2014). Assume that Y be a fixed set. A PFS X on Y is defined in the following mathematical form:
Definition 2 (Wu and Wei, 2017). For a PFN ς=(μς,νς), the modified score and accuracy functions are as
Definition 3 (Yager, 2013, 2014). Let ς=(μς,νς),ς1=(μς1,νς1) and ς2=(μς2,νς2) be three PFNs. Then, the different operations on PFNs are given by
ςλ=((μς)λ,1−(1−νς2)λ),λ>0.
Definition 4 (Zhang and Xu (2014). Let ς1=(μς1,νς1) and ς2=(μς2,νς2) be three PFNs. Then a distance measure between ς1 and ς2 is given as follows:
Next, this section develops the PF-entropy-RS-MARCOS method for solving MCDM problems from a Pythagorean fuzzy perspective. This method involves the following steps (see Fig. 1:
Step 1: Generate a “linguistic decision-matrix (LDM)”.
A set of l DEs A={a1,a2,...,al} has to choose the most optimal alternative from a set of m optionsH={h1,h2,...,hm} with respect to n criteria/attributes U={u1,u2,...,un}, respectively. Assuming the ambiguity of human behavior and lack of information about the options, the DEs give “linguistic values (LVs)” to assess his/her opinion on option hi over an attribute cj. Assume that Z(k)=(ξij(k))m×n;i=1(1)m,j=1(1)n be the LDM provided by set of DEs, in which ξij(k) refers to the linguistic performance value of an option given by kth expert.
Step 2: Compute the weights of DEs
The formula for the computation of DEs’ weights is given as follows:
Here, ϖk≥0 and ∑k=1lϖk=1.
Step 3: Make the “aggregated PF-decision matrix (A-PF-DM)”.
The A-PF-DM is created using the “PF-weighted averaging (PFWA)” operator and we get A=(ξij)m×n, where
Step 4: Proposed PF-subjective and objective weighting approach.
This process involves three cases:
Case I: Objective weights by entropy formula.
To derive the objective weights of criteria, the entropy model is extended under the PFS environment as
where,Case II: Subjective weights using the rank sum (RS) model.
Here, the use of the subjective weighting system helps reflect the decision-makers’ thoughts and intrinsic values (Stillwell et al., 1981; Hezam et al., 2022). The RS model aids the DEs in allocating the ranking values to the selected criteria. Eq. (9) can be used to find the weight (wjs)
where rj signifies the rank of jth criterion, j = 1(1)n.Case III: Obtain the combined weight of criteria using the PF-entropy-RS model.
Based on objective and subjective weights, the combined weighting formula is given as follows:
where γ∈[0,1]is the strategic factor to show the variation of attribute weights.Step 5: Construct the “normalized A-PF-DM (NA-PF-DM)
The NA-PF-DM R=(ςij)m×n is determined by A=(ξij)m×n,
Step 6: Estimate the reference points.
We compute the “Pythagorean fuzzy-ideal solution (PF-IS)” and “Pythagorean fuzzy anti-ideal solution (PF-AIS)” with the use of the following expressions:
Step 7: Calculate the “weighted NA-PF-DM (WNA-PF-DM)”.
The WNA-PF-DM Nw=(ς⌢ij)m×n is determined, where
Step 8: Evaluate the score values of the weighted sum of each option
In Eq. (15), S(ς⌢ij) represents the score values of each element of the WNA-PF-DM.
Step 9: Evaluate the “utility degree (UD)” of option
Step 10: Identify the “combined utility function (CUF)”.
The CUF is the compromise solution of alternatives associated to the PF-IS and PF-AIS. Thus, the CUF of alternatives is defined by
Step 11: Rank the alternatives based on the CUFs. The appropriate option has the maximum CUF value.
The higher education sector is undertaking “technologization” worldwide (Lea & Goodfellow, 2007). This has different consequences, for example, the reduction of the governmental financial support of higher education, the increase of competition due to globalization, and the affordances of digital technologies (Jones & O'Shea, 2004). The reason is that it is widely acknowledged that computing and related technologies are able to enhance the quality of things, especially with the wide adoption of technology as a dominant power. It has been particularly reinforced when it is associated with a profusion of research disseminating it through searching for evidence indicating the revolutionary effects of technologies on education (Bigum et al., 2015). Especially, this is the case when considering the centralized higher education system in China. The Chinese government has consistently put great importance on the mission of digitally transforming the (higher) education in this country. For instance, the Higher Education Law of the People's Republic of China and the Education Law of the People's Republic of China oblige the government to give its support at all levels to the acceptance and implementation of technology in education, which includes constructing an IT infrastructure, giving access to and sharing of high-quality educational resources, and strengthening the process of teaching and administration. In 1998, the Chinese Ministry of Education (MOE) publicized an Action Plan for the invigoration of education in its path toward the 21st century (MOE, 1998). The plan called for different things, including using the Modern Distance Education Initiative to employ modern IT in the best way and gain equal access to educational resources. The latter was particularly important to China since this country still suffered from an uneven distribution of educational resources. Then, one year later, the Central Committee of the Chinese Communist Party and State Council broadcasted the decision made in regard to deepening the reformation of the education sector and the promotion of quality-oriented education. Article 15, the law enacted in this regard, indicates the strong support given by the government to the process of digitalizing education. Moreover, some years before that, in 2010, the government had also published the Outline of China's National Plan for Medium and Long-term Educational Reform and Development (2010–2020). Chapter 19 of the Plan emphasizes the need for the acceleration of digital transformation, which includes the acceleration of digital infrastructure construction and the modernization of contents, methods, and means of instruction; the development, implementation, and sharing of high-quality educational sources on a greater scale; rethinking the teaching, improvement of pedagogies, and enhancement of the teaching effectiveness by means of IT; the encouragement of learners for the utilization of IT to help them improve their active learning, autonomous learning, and problem-solving; and the construction of an education information management system at a national level for the improvement of the management effectiveness and also making decision-makers more informed. Accordingly, MOE (2012) issued its Ten-Year Development Plan for Educational Digitalization (2011–2020), setting the following goals: 1) to narrow the digital divide, 2) to accelerate digitalization in vocational education, 3) to upgrade the community service capacity, 4) to enhance the education management efficiency, 5) to share high-quality educational resources, 6) to enhance the lifelong learning system, 7) to support the professional development, 8) to encourage the integration of IT and higher education, and 9) to make sure of sustainability.
Up to 31 May 2017, a total of 2914 HEIs existed in China, which included 2631 conventional campus-based HEIs as well as 283 adult HEIs. Seventy-five HEIs were affiliated with MOE, around 30 HEIs were affiliated with other ministries, and the remaining HEIs were affiliated with the provincial governments or local education authorities. The 13th five-year development plan (2016–2020) of the 75 universities directly under MOE was selected in this study as the sample since they are completely accessible at the official website of MOE of China. Statistics show that the number of internet users in this country has reached 649 million. In such conditions, the education sector has been ‘informationized’ and digitalized, which has possessed the following main characteristics. A total of 530,000 educational institutions exist in China, with around 270 million registered students. In 2014, the online education value in this country exceeded USD 13 billion, and it was expected to reach up to USD 28.27 billion in 2015. Such an enormous market fascinated many Internet companies such as Alibaba, Baidu, and Tencent, as well as many education service integrators, education equipment and software firms, and educational institutes.
However, the objective of the present work is to identify the competencies of the future workforce for digital technologies implementation in higher education. In this regard, in the first stage, the comprehensive literature review is done to identify the main competencies of the future workforce for digital technologies implementation. In the next stage, we have invited 46 teaching and academic staff recruited from different institutions. The institutions were invited to participate in this study via email. The anonymity/confidentiality of the participants was also clearly stated. Staff were asked to complete a questionnaire comprising a total of 50 questions. In addition, some pieces of necessary background information were gathered through the questionnaire. Among all, 37 staff preferred to complete the questionnaire in online mode, whereas nine staff preferred to complete the print version following a workshop held at their institutions. Results of this steps of the study indicated that 25 the competencies of the future workforce for digital technologies implementation in higher education are important that including; u1: IT/ ICT competencies, u2: Innovation capability, u3: Working in interdisciplinary environments, u4: Analytical skills, u5: Implementation of ideas in the shop floor, u6: Conflict solving self-and time management, u7: Social intelligence, u8: Creativity, u9: Exchange of opinions and ideas, u10: Commitment to lifelong learning, u11: Ability to communicate about complex problems, u12: Generic knowledge for production and technologies processes, u13: Mindset for building and maintaining networks of experts, u14: Personal flexibility, u15: Problem solving, u16: Data and information processing, u17: Collaboration skills, u18: Decision making, u19: Teamwork, u20: Value-driven management style, u21: Ability to solve complex concepts for realistic work packages use by appropriate people, u22: Ability to interact modern interfaces, u23: Encouragement taking new routes, u24: Understanding of interrelations technological components and u25: Process understanding.
In general, research design depends on the nature of the research problem at hand (Creswell, 2002); for the purpose of the current study, a survey was selected as the method that could meet the research objectives. Practically, the qualitative approach, as opposed to the quantitative one, is typically adopted to gain a deep understanding of a certain social condition, role, event, group, or interaction within a wider societal context (Cohen et al., 2002; Mills & Gay, 2019). This approach helps to take into consideration ‘the state, properties, and characteristics of a certain phenomenon (Labuschagne, 2003). The qualitative approach aids the researchers, who are thought of as insider participants, in comprehending the complexity of viewpoints, which may even include various ‘voices’ that are hardly heard or even totally ignored. In this stage, a questionnaire is designed to include 25 competencies of the future workforce for digital technologies implementation. To evaluate these 25 identified competencies, we sent questionnaires to 10 decision experts from the academic in higher education institutions via email invitations, and finally, we could collect the data from four decision experts. To analyze the 25 identified competencies, this study proposed a novel PF-entropy-RS-MARCOS method. The implementation of the PF-entropy-RS-MARCOS method is discussed as follows:
Steps 1–3: Table 1 shows the linguistic performance values of DEs and their corresponding PFNs (Rani et al., 2020a,b). Based on Table 1 and Eq. (4), we have computed the DEs’ numeric weights in Table 2. According to DEs’ opinions, we have constructed the LDM in Table 3. Then the A-PF-DM is formed using Tables 1, 2, and Eq. (5).
Step 4: With the use of formulae (6)-(8), we have derived the objective weights of criteria for the competencies of the future workforce for digital technologies implementation in higher education wjo= (0.0417, 0.0415, 0.0444, 0.0365, 0.0413, 0.0361, 0.0402, 0.0465, 0.0388, 0.0311, 0.0405, 0.0400, 0.0347, 0.0351, 0.0395, 0.0531, 0.0398, 0.0517, 0.0399, 0.0413, 0.0387, 0.0342, 0.0394, 0.0397, 0.0346).
Performance ratings of alternatives over criteria and DEs regarding the LVs.
The LDM of each option by DEs to the competencies of the future workforce for digital technologies implementation.
Using the formula (9), the subjective weights of criteria are determined for the competencies of the future workforce for digital technologies implementation in higher education (see Table 5).
Based on the combination of objective and subjective weights, the final weights of criteria are computed for γ=0.5, given as wj = (0.0470, 0.0315, 0.0299, 0.0228, 0.0222, 0.0550, 0.0555, 0.0325, 0.0379, 0.0394, 0.0233, 0.0369, 0.0296, 0.0329, 0.0482, 0.0596, 0.0437, 0.0428, 0.0484, 0.0537, 0.0394, 0.0556, 0.0505, 0.0260, 0.0388).
Here, Fig. 2 shows the weight values of different competencies of the future workforce for digital technologies implementation in higher education g with respect to the goal. Data and information processing (u16) has emerged to be the most important competencies of the future workforce for digital technologies implementation in higher education. Ability to interact modern interfaces (u22) is the 2nd most important competency of the future workforce for digital technologies implementation in higher education. Social intelligence (u7) has secured 3rd position, Conflict solving self-and time management (u6) has secured 4th position, Value-driven management style (u20) has 5th most important competencies of the future workforce for digital technologies implementation in higher education, and others are considered crucial competencies of the future workforce for digital technologies implementation in higher education.
Step 5: Since all criteria are beneficial-type criteria, thus, there is no need to transform the A-PF-DM into normalized form.
Step 6: From Eq. (12)-Eq. (13) and Table 4, the PF-IS and the PF-AIS for the competencies of the future workforce for digital technologies implementation in higher education are obtained as follows:
Table 4.The A-PF-DM for competencies of the future workforce for digital technologies implementation.
Table 5.Weights of competencies of the future workforce for digital technologies implementation using the RS method.
αj+= {(0.741, 0.412, 0.530), (0.628, 0.518, 0.580), (0.724, 0.428, 0.540), (0.665, 0.482, 0.571), (0.764, 0.392, 0.513), (0.664, 0.485, 0.569), (0.533, 0.598, 0.599), (0.754, 0.405, 0.518), (0.699, 0.461, 0.547), (0.696, 0.457, 0.554), (0.654, 0.497, 0.570), (0.675, 0.476, 0.564), (0.624, 0.522, 0.582), (0.683, 0.482, 0.549), (0.714, 0.452, 0.535), (0.696, 0.457, 0.554), (0.558, 0.578, 0.596), (0.725, 0.430, 0.539), (0.726, 0.434, 0.533), (0.587, 0.553, 0.591), (0.589, 0.556, 0.587), (0.631, 0.532, 0.564), (0.692, 0.468, 0.550), (0.660, 0.499, 0.562), (0.605, 0.546, 0.580)}
αj+= {(0.430, 0.681, 0.593), (0.313, 0.777, 0.546), (0.567, 0.579, 0.585), (0.470, 0.668, 0.577), (0.538, 0.592, 0.600), (0.401, 0.702, 0.589), (0.366, 0.713, 0.598), (0.469, 0.673, 0.572), (0.560, 0.579, 0.593), (0.541, 0.594, 0.595), (0.386, 0.702, 0.598), (0.417, 0.677, 0.607), (0.485, 0.652, 0.583), (0.569, 0.567, 0.596), (0.547, 0.576, 0.608), (0.370, 0.708, 0.602), (0.372, 0.710, 0.598), (0.386, 0.715, 0.583), (0.383, 0.712, 0.589), (0.393, 0.704, 0.591), (0.451, 0.675, 0.584), (0.449, 0.664, 0.598), (0.497, 0.616, 0.611), (0.426, 0.702, 0.570), (0.387, 0.726, 0.568)}.
Step 7: According to Eq. (14) and Table 4, the weighted NA-PF-DM for the competencies of the future workforce for digital technologies implementation in higher education is created and given in Table 6.
Table 6.The weighted NA-PF-DM of each option.
Step 8: Using Eq. (15) and Table 6, the score values of each alternative, PF-IS, and PF-AIS for the competencies of the future workforce for digital technologies implementation in higher education are determined and given in Table 7.
Table 7.Score values of WNA-PF-DM of each option.
Steps 9–11: From Eqs. (16) and (17), we estimate the utility degrees, CUFs, and preference order of alternatives which are given in Table 8. Hence, the prioritization of options is h4≻h1≻h3≻h2≻h5, and the higher education option-IV (h4) is the best choice with maximum CUF for the competencies of the future workforce for digital technologies implementation in higher education.
The present subsection presents the sensitivity investigation with respect to a parameter γ. The changing values of γ aid in evaluating the approach sensitivity level, changing from weight values of criteria from the subjective to the objective assessments of options and their preferences.
Table 9 and Fig. 3 present the results obtained from the sensitivity investigation. For γ = 0.0 to γ = 0.5, we obtained the ranking order as h4≻h3≻h1≻h2≻h5; for γ = 0.6 to γ = 0.9, we obtained h4≻h1≻h3≻h2≻h5 and for γ = 1.0, we obtained h4≻h1≻h2≻h3≻h5. Thus, the alternative h4 has secured the best rank for all the values of γ, while the h5 has the last rank for each value of γ. Accordingly, the present PF-entropy-RS-MARCOS has satisfactory stability with respect to many values of parameter γ. Table 9 illustrates that the developed PF-entropy-RS-MARCOS method can produce preference results in various strategic parameters with both stability and flexibility. Such characteristic is of high significance to the MCDM processes and decision-making reality.
Results of the PF-entropy-RS-MARCOS method w.r.t. different values of γ.
This section compares the results obtained by the PF-entropy-RS-MARCOS method and some of the existing methods, such as the PF-TOPSIS model (Zhang & Xu, 2014) and PF-WASPAS (Rani et al., 2020b) for solving MCDM problems under PFSs context as follows:
PF-TOPSIS modelSteps 1–4: Follow the steps of the PF-TOPSIS method.
Step 6: Derive the degrees of distances of options from PF-PIS and PF-NIS.
In accordance with Eq. (3), estimate the degree of distance D(hi,ϕ+) among the option hi and the PF-PIS ϕ+.
and the degree of distance D(hi,ϕ−) among the options hi and the PF-NIS ϕ− is given as follows:Step 7: Compute the relative closeness index (CI)
C(hi)=D(hi,ϕ−)D(hi,ϕ+)+D(hi,ϕ−),i=1(1)m. (20)
Based on the values of CI, the most suitable candidate and the prioritization order of all options are estimated. The maximum value of C(hk) determines the most appropriate choice.
Using Eq. (18)-Eq. (20), the overall computational results and prioritization order of the higher education options to the competencies of the future workforce for digital technologies implementation in higher education (see Table 10). Thus, the most suitable higher education option is h4 to the competencies of the future workforce for digital technologies implementation in higher education. The priority order of options is h4≻h3≻h1≻h2≻h5 to the evaluation of the competencies of the future workforce for digital technologies implementation in higher education.
PF-WASPAS modelThe PF-WASPAS method is implemented to handle the decision-making problem. The description of PF-WASPAS method is given as follows:
Steps 1–5: Follow the proposed model.
Step 6: Utilize the weighted sum model (WSM) Ci(1) and weighted product model (WPM)Ci(2) in the following expression
Step 7: Determine the measure of WASPAS for each option as
Step 8: Rank the options according to WASPAS measures.
We have implemented the PF-WASPAS method in the given case study to select a suitable alternative to the competencies of the future workforce for digital technologies implementation in higher education. The computational results are shown in Table 11.
The UD of option to the competencies of the future workforce for digital technologies implementation.
As a whole, the benefits of the PF-entropy-RS-MARCOS method over the extant methodology are given (see Fig. 4):
- ■
In the developed method, the subjective weights of attributes are obtained by the PF-RS method, and the objective weights of the competencies of the future workforce for digital technologies implementation in higher education are computed by entropy-based method, whereas in PF-WASPAS, only objective weights of criteria are obtained by entropy and divergence measure-based weighting procedure and in PF-TOPSIS, the criteria weights are chosen arbitrarily.
- ■
In Zhang and Xu (2014), the measure of distance is computed between the overall attribute value of an alternative hi and the PF-IS and the PF-AIS to describe the CI of each option on the given attributes. The PF-PIS and PF-NIS could be considered two benchmarks against which the performance of the alternatives on each attribute could be assessed. Remember that the two above-mentioned benchmarks are too unrealistic to be achieved practically. On the other hand, it should be noted that the proposed PF-entropy-RS-MARCOS uses the aggregated compromise algorithm with different aggregation strategies to acquire a compromise solution. The MARCOS tool comprises (i) considering “Pythagorean fuzzy ideal solution (PF-IS)” and “Pythagorean fuzzy anti-ideal solution (PF-AIS)” as reference points, (ii) establishing the relationship with options and PF-IS/PF-AISs, (iii) describing the “utility degree (UD)” of each option in association to PF-IS and PF-AISs.
Nowadays, companies and governments are progressively more aware to fill the gap between the existing and required DCs of the workforce to successfully cope with the challenges that may arise in the future digitalized workplaces. However, the literature still lacks a holistic framework and a comprehensive definition of DCs. The current paper attempted to suggest an inclusive framework of the DC concept with a focus on applications at work. To this end, the present study combined different methods to provide an integration of various perspectives on DCs. This paper extensively reviewed the relevant literature in terms of the definitions and frameworks of DCs, which could be applied to work. In addition, the current study provided significant insight into the construction of DCs at work through the provision of an overview of the previously proposed definitions and methodologies in this regard. The review of the existing confirmed the absence of scientific research on adults’ DC, and also it was revealed that the work context had been totally neglected. The large variety of terms and constructed frameworks reveals an interest in DCs in various settings, e.g., education, media, and politics. The present paper addressed the science-practice gap through the integration of various perspectives in this regard. For that reason, in addition to the systematic literature review, eleven half-structured interviews were held with a number of practitioners from the work context. Nevertheless, the major aim of the present paper is to identify the competencies of the future workforce for digital technologies implementation in higher education. In this regard, in the first stage, the comprehensive literature review is done to identify the main competencies of the future workforce for digital technologies implementation. Therefore, fifty competencies of the future workforce for digital technologies implementation are identified. In the next stage, we have invited 46 teaching and academic staff recruited from different institutions. The participant institutions were invited via email. The email consisted of information about the aim and process of the study. The participants were assured of their confidentiality and anonymity. A questionnaire comprising 50 items was sent to each participant to be completed. The results of these steps of the study indicated that 25 criteria are the key competencies of the future workforce for digital technologies implementation in higher education.
To analyze, rank and evaluate the main to identify the competencies of the future workforce for digital technologies implementation in higher education, this study introduced a hybrid method from the Pythagorean fuzzy perspective. A novel decision-making approach based on the PF-entropy-RS and PF-MARCOS methods, termed the PF-entropy-RS-MARCOS method, was introduced for the assessment of the major competencies of the future workforce for digital technologies implementation in higher education. To rank main competencies of the future workforce for digital technologies implementation in higher education, the PF-entropy-RS method was used, and for the calculation of the preference order of different higher education institutes to the evaluation of the competencies of the future workforce for digital technologies implementation in higher education, the PF-MARCOS method was employed. To validate the results obtained in the current work, a comparative study was also performed with the use of different models existing in the literature. Also, the sensitivity analysis of criteria weights was implemented to authenticate the proposed model's robustness and applicability.