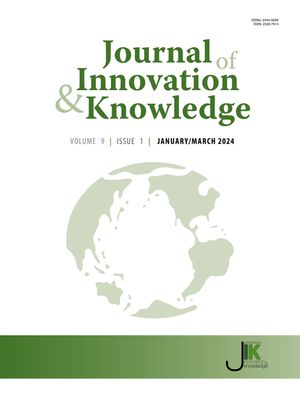
Edited by: Brij B. Gupta, Kwok Tai Chui
More infoInnovation is directly related to development and competitiveness, and collaborative innovation in universities is an important way to give full play to the advantages of knowledge accumulation. However, because there are multiple party participants, many categories and quantities of data indicators and high dimensions of evaluation and comparison and because the process of acquiring knowledge is constantly evolving, the construction of an evaluation method and the corresponding system is a key challenge. Therefore, a performance evaluation method based on an improved order relation analysis (G1)-Criteria Important Through Intercriteria Correlation (CRITIC) and the Technique for Order of Preference by Similarity to the Ideal Solution (TOPSIS) method for collaborative innovation is proposed and discussed. Then, we design and implement a performance evaluation system for university collaborative innovation. Using data from 73 collaborative innovation centers in Jiangsu from 2015 to 2019, a basic data set is constructed to conduct an empirical analysis of performance evaluation. Furthermore, the evaluation results are compared with those of existing comprehensive evaluation methods. The experimental results show that the proposed evaluation method can objectively and effectively evaluate the performance of collaborative innovation centers. In terms of knowledge, this study draws the following key conclusions. The overall level of construction of collaborative innovation centers in Jiangsu Province is relatively high, but the overall development is unbalanced, and the level of performance of different types of collaborative innovation centers is significantly different.
For all industries, innovation constitutes the core of their abilities (Aslam, Saleem, Khan & Kim, 2021), and the innovativeness, speed, and frequency of innovations are important success factors for enterprises and universities (Hilmersson & Hilmersson, 2021). In particular, knowledge-intensive industries compete primarily in terms of their capacity to innovate, and they thrive on cutting-edge knowledge, which drives both research and innovation (Okatan, 2012; Gloet & Samson, 2016). In this context, focusing on collaborative innovation in universities holds great significance. Collaborative innovation in universities is an innovation mechanism that is complemented and co-operated by multiple units with different attributes. It is also an important and direct way to give full play to the cumulative advantages of universities and to deepen industry–university–research collaboration. Since the implementation of China's “2011 Plan”, the state, local governments, universities and key enterprises have been increasingly investing in the construction of collaborative innovation centers and paying more attention to collaborative innovation performance. The level of collaborative innovation performance has an important impact on breakthrough solutions to major theoretical problems at the forefront of international science, the cultivation of high-level innovative talent, the research and development of highly sophisticated core technologies in industry, and the enhancement of the independent innovation capability of key enterprises in regional pillar industries. Continuous research on collaborative innovation performance in universities can not only enrich and expand people's understanding of the operation mechanism of collaborative innovation in colleges and universities at the theoretical level but also guide participating parties to better grasp the inner laws and key elements of collaborative innovation activities at the practical level.
Traditionally, innovation performance is measured in terms of economic value for the developing firm (Witell, Snyder, Gustafsson, Fombelle & Kristensson, 2016). However, the method of evaluating the collaborative innovation ability of universities is different from that of enterprises (Lu & Tseng, 2010). From the perspective of the collaborative innovation data life cycle (Polyzotis, Roy, Whang & Zinkevich, 2018), the data generated (Cavanillas, Curry, & Wahlster, 2016) come from the timely uploaded data of many colleges and universities, and there are many categories and quantities in the data (Chaudhary, Gupta, Chang, Nedjah & Chui, 2021). Then, multiple party participants need to use a variety of analysis, sharing and evaluation methods to capture the knowledge (Gupta & Quamara, 2020). For example, the education office will pay attention to abnormal data and the overall development situation in different stages; universities are more concerned about the comparison of data indicators and evaluation rankings. The data curation (Stonebraker & Xu, 2013) of institutionalized collaborative innovation over time will continuously organize and utilize incremental data to analyze new changes in individual participants and to gain previously unobserved knowledge. Clearly, the process of acquiring knowledge from the collaborative innovation data life cycle is constantly evolving and complex, and the construction of an evaluation method and the corresponding system is a key challenge (Jennex & Durcikova, 2014).
At present, some research on the evaluation of collaborative innovation performance in universities has obtained good application results. However, given that collaborative innovation in universities has a specific data life cycle and the correlation between evaluation metrics is implicit and difficult to quantify and organize, it is still worth exploring how to approach this issue scientifically and comprehensively. Constructing and comparing diverse evaluation models, tools and systems, further collecting feedback on all aspects, and implementing timely and relevant indicators for adjustment and optimization are some of the keys of research on this topic. Therefore, in this paper, we propose a novel method based on an improved order relation analysis (G1)-Criteria Important Through Intercriteria Correlation (CRITIC) method to evaluate the performance of university collaborative innovation. Incorporating the collaborative innovation data life cycle, we present the implementation of this method as a feasible system for collaborative innovation evaluation, presenting diversified analysis results and verifying the proposed method. First, based on a data set of collaborative innovation centers in universities in Jiangsu Province, the corresponding performance evaluation index system is established through data analysis. Second, the weight of each index is calculated by using the combination weighting method of the improved G1 method (Zhu, 2017; Gong & Liu, 2019) and CRITIC method (Diakoulaki, Mavrotas & Papayannakis, 1995). Then, the Technique for Order of Preference by Similarity to the Ideal Solution (TOPSIS) (Zhao & Tang, 2019) is used to build the performance evaluation model, compute the performance evaluation scores and rank the universities under evaluation. The implementation of the evaluation system is performed using Python (Kong, Siauw & Bayen, 2020) and MongoDB (Bradshaw, Brazil & Chodorow, 2019). Data from 73 collaborative innovation centers in universities in Jiangsu Province from 2015 to 2019 are analyzed and verified. The constructed evaluation system can provide more scientific and intuitive evaluation results for measuring implementation effectiveness in universities. The results of the innovation performance evaluation can have a positive impact on improving the innovation capability of university collaborative innovation centers and promoting the practice of university collaborative innovation.
The rest of this paper is organized as follows: Section 2 introduces the research work related to this paper. Section 3 introduces the process of constructing the performance evaluation model of collaborative innovation in universities. In Section 4, incorporating the performance evaluation system for collaborative innovation centers, the analysis results are presented, and the improved G1-CRITIC method is compared with other classical comprehensive evaluation methods. Finally, Section 5 summarizes and discusses future research prospects.
Related worksScholars in China and elsewhere have conducted research on evaluation systems and collaborative innovation performance from different perspectives, and they have used various methods to analyze and evaluate collaborative innovation data, such as cloud models, fuzzy evaluation methods, data envelopment analysis (DEA) and hybrid models.
Xu Jia (Xu, Li, Li & He, 2020) constructed a performance evaluation index system for collaborative innovation by consulting relevant experts, conducting a questionnaire survey and carrying out proportional scale scoring. They processed data based on the particle swarm optimization (PSO)-analytic hierarchy process (AHP) method and established a performance evaluation model. Finally, the actual work and performance results of teachers in collaborative innovation centers in universities were investigated, and the assessment model was applied to evaluate their actual work performance, verifying the rationality and feasibility of the proposed model. Jiang Xinghua (Jiang, Wang & Fan, 2020) constructed a performance evaluation system, adopted the fuzzy evaluation method for comprehensive evaluation, and made suggestions on how to obtain excellent results in the performance evaluation of collaborative innovation center construction in universities. Li Yongzhou (Li, Quan & Wu, 2018) evaluated the performance of 22 collaborative innovation centers in Hubei Province by constructing a fuzzy comprehensive evaluation model and using the Delphi method and expert scoring to assign weights. Zhong Yuting (Zhong, 2016) used a strategic map evaluation model to implement a performance evaluation index system for an industry-oriented collaborative innovation center and then analyzed the performance evaluation and results of four sample centers by employing the performance evaluation method used with the industry-oriented collaborative innovation center based on a normal cloud model. Li Aibin (Li, Jiang & Xia, 2016) proposed a performance evaluation index system for collaborative innovation centers involving three dimensions, including collaborative innovation output performance, collaborative innovation behavior performance and a collaborative innovation environment, and designed a cloud model to evaluate the performance of industrial collaborative innovation centers through examples. Yildiz et al. (Yildiz, & Ahi, 2022) proposed a hybrid model of strategic and operational indicators incorporating four evaluation methods. The analytic network process (ANP), TOPSIS and Decision-Making Trial and Evaluation Laboratory (DEMATEL) were proposed as an integrated approach to support innovative decision-making for supply chain performance management.
Establishing a scientific and reasonable evaluation index system, including index selection and weight assignment, is the key to conducting evaluation. The works above mainly built an evaluation index system by superimposing an expert screening index on a subjective weighting method. The subjective weighting method assigns weights to indicators based on the experience of experts. It usually requires questionnaires to be completed by many experts, which is very costly and is easily directly affected by the opinions of different experts. Additionally, it is difficult to reproduce the performance evaluation results.
Zhu Jinlong (Zhu, Zhu & Song, 2018) used super-efficiency DEA (SE-DEA) and principal component analysis (PCA) to analyze the construction of collaborative innovation centers in universities based on the rate of utilization of input resources and the efficiency of scientific research output, taking 27 industrial collaborative innovation centers as examples. Based on a PCA-stochastic frontier analysis (SFA) model, the same authors (Zhu, Zhu & Song, 2019) further studied the construction efficiency of 59 collaborative innovation centers approved to be built in four categories, i.e., frontier science, cultural heritage, business and industry, and regional development, in terms of the completion of major collaborative tasks, disciplinary construction, scientific research, personnel training, service to society and international cooperation and exchange. Jiang Mingye (Jiang, 2019) utilized PCA and a three-stage DEA model to analyze the comprehensive efficiency, scale efficiency and technical efficiency of universities in Jiangxi Province. Based on a DEA-Tobit model, Liu Tianzuo (Liu & Zhang, 2019) empirically studied the performance (input‒output efficiency) of 35 collaborative innovation centers and its influencing factors. Chen Ying (Chen, 2018) used PCA to process the input‒output index and then constructed a performance evaluation model of the collaborative innovation center for cultural heritage at Jiangsu University based on a basic DEA model and SE-DEA to empirically analyze and comprehensively evaluate the performance of this center. Zhao Leiying (Zhao, 2016) first constructed a performance evaluation system for industry–university–research collaborative innovation based on factor analysis, then summarized the influencing factors of industry–university–research collaborative innovation performance based on grounded theory, and finally built a model based on system dynamics to analyze the mechanism of influencing factors. Aytekin et al. (Aytekin, Ecer, Korucuk & Karamaşa, 2022) evaluated the innovation efficiency of EU member states and candidate countries based on the DEA-Efficiency Analysis Technique with Input and Output Satisficing (EATWIOS) multicriteria method. The results showed that the Netherlands, Germany and Sweden were the most important countries in the world for innovation efficiency. At the same time, some suggestions were proposed to address the shortcomings of noninnovative countries.
The literature above mainly used an objective weighting method to weight evaluation indexes. DEA has become the choice of many researchers who make full use of its extremely valid and mature characteristics to evaluate university performance and rankings. However, there may be some illogical situations in the actual calculation process, as subjective factors are completely ignored. For example, as the greatest limitation of the method represented by DEA, the use of linear programming to calculate has certain restrictions in terms of the observed sample data. Furthermore, linear programming sometimes needs to discard some sample values, which will directly affect the stability and accuracy of the analysis results. In addition, the research work above neglects to consider the evaluation effect, the reproduction of results and the comparison of differences under different comprehensive evaluation methods.
With the development of some intelligent technologies, the construction of evaluation systems is no longer limited to statistics and expert scoring methods (Hu, Gaurav, Choi & Almomani 2022). This kind of work is combined with cutting-edge technologies such as machine learning or graph theory to find patterns in large amounts of data and conduct generalized data analysis (Kar, 2022; Pan, Yamaguchi, Kageyama & Kamilin, 2022). However, due to their poor interpretability and complex model design, evaluation systems incorporating intelligent technologies have not been widely applied in the field of performance evaluation (Chui, Gupta & Vasant, 2021).
In summary, at this stage, there are still two key aspects of research on performance evaluation systems for collaborative innovation in colleges and universities that need to be improved. (1) Appropriate and diversified methods of analyzing and evaluating the performance of collaborative innovation centers in universities still need to be explored, and there is a lack of a corresponding comprehensive evaluation system and visualization platform to analyze and evaluate historical data. (2) There is a lack of tools to compare the evaluation performance and results under different comprehensive evaluation methods. To address these key issues, this paper constructs a multicriteria comprehensive evaluation model based on an improved G1-CRITIC method and the TOPSIS method. Furthermore, it implements a multifunctional visualization system for collaborative innovation performance evaluation in universities to present the results and verify the usability of the method. In addition, the method and system have been used by several participants to acquire domain knowledge of collaborative innovation.
Comprehensive evaluation method based on an improved G1-CRITIC methodThis section describes the main steps used by the proposed evaluation method. First, in Section 3.1, we introduce the data used and how they are prepared for further utilization in the process. Then, in Section 3.2, how to build the evaluation index system is described. Subsequently, in Section 3.3, the improved metric for the proposed evaluation method is presented. In Section 3.4, we explain how the final ranking score is computed. The main process of evaluating institutionalized collaborative innovation is shown in Fig. 1.
Data source and preprocessingThe collaborative innovation data adopted come from the uploaded data of colleges and universities from 2015 to 2019. Because the units of various evaluation indexes are different, before calculating the overall performance evaluation value, the evaluation value of each factor is dimensionless, and min-max normalization is specifically adopted as shown in Eq. (1).
Construction of the evaluation index system for collaborative innovation centers in universitiesThe design of an index system should focus on the three principles of differential evaluation, three-dimensional evaluation and comprehensive evaluation (Zhao, 2014). The index used to measure innovation capability should be multidimensional because a single-dimensional analysis may generate measurement bias in organizational innovation (Taques, López, Basso & Areal, 2021). Therefore, the evaluation index system for collaborative innovation centers constructed here fully considers the orientation of innovation and development. The system takes 8 aspects as the primary indicators of the evaluation index system: the composition of capital investment, the composition of current employees, the introduction of outstanding talent, the acquisition of major awards, the paper output, the patent output, international exchanges and cooperation, and service and contribution to society. Additionally, it selects 22 typical secondary indicators from the 8 different primary indicators above based on the three principles. The indicators are listed in Table 1 below, covering the existing major elements of university collaborative innovation center data, including capital investment, personnel training, international cooperation and scientific and technological output. Furthermore, the indicators reflect the input‒output situation of participating subjects, such as colleges and universities, as comprehensively as possible.
Index system.
A combined weighting method is usually a combination of various weighting methods, and it combines subjective and objective aspects to calculate weights, considering the diversity of evaluation indicators and some hidden relationships. Therefore, from a practical perspective, a combined weighting method is more suitable for the performance evaluation of collaborative innovation centers in colleges and universities. Specifically, this paper first considers adopting a double-layer combined weighting method based on the G1 method and CRITIC method. It adds some subjective factors that are easier to judge based on an objective weighting method to control the illogical situation of the objective weighting method. Among them, the G1 method is an improvement on the AHP. Compared with the AHP, the G1 method has been widely studied by scholars in China and elsewhere because of its simple calculation and lack of need for consistency testing. The original G1 method needs not only the order relation between indicators but also the relative importance scale between adjacent indicators, which is difficult to determine in the process of practical application. For example, it can be confirmed that the number of newly added academics is more important for universities than the number of newly added Changjiang scholars; however, the degree of importance is often difficult to judge. Therefore, this paper adopts a hybrid cross-weighting method based on the coefficient of variation and the G1 method.
First, the order relation between secondary indicators is determined by referring to experts’ opinions. Methodologically, the coefficient of variation of each index of a collaborative innovation center is calculated. The importance of each index is determined by the ratio of the coefficient of variation between adjacent indexes. In the process of practical application, the ratio of the coefficient of variation between adjacent indicators is less than 1. The conventional practice is to set the relative importance scale to 1; that is, two adjacent indicators are equally important, which is not in line with the actual situation because there is an obvious order relation between indicators. To solve this problem, a weight difference benchmark is determined between adjacent indexes, and the weight benchmark is set to 1.2, which is usually regarded as the degree indicating "slightly important". Based on this benchmark, the relative importance between adjacent indexes is calculated by combining the ratio of the coefficients of variation. The improved G1 method avoids the problems of randomness and subjectivity in determining relative importance and introduces the weight difference benchmark, which makes the determination of weights more reasonable and objective. The specific calculation steps are as follows.
The first step is to determine the weight order among the indicators based on expert opinions and to construct the order relation among the indicators, which is recorded as shown in Eq. (2):
The second step is to determine the value of the coefficient of variation of the evaluation index.
The standard deviation of the calculated index is calculated according to Eq. (3):
The coefficient of variation of the evaluation index is calculated as Eq. (4):
The third step is to determine the degree of importance between adjacent indexes based on the ratio of the coefficients of variation of the evaluation indexes according to Eq. (5):
The fourth step is to determine the final weight of each index based on the G1 method.
Based on the relative importance scale obtained in the previous step, the weight of index n is obtained according to Eq. (6):
Then, the subsequent weight values adjacent based on the weight of ωn are calculated according to Eq. (7):
Through the improved G1 method described above, the weight of each index group, that is, the secondary weight can be obtained. Next, the weight between the primary indexes is calculated based on the CRITIC method, and the final weight of each index is determined. The CRITIC method is a method for determining index weights based on contrast intensity and the conflict between indexes. Compared with the entropy weight (EW) method (Yuan & Wang, 2021), it takes into account the degree of difference within the same index and the correlation between different indexes, and it has been widely used. The performance indicators of collaborative innovation centers in Jiangsu universities do not exist in isolation, and there is a strong correlation between them; thus, the CRITIC method is applicable for this situation. The CRITIC method not only measures the degree of difference within the same index based on the standard deviation but also uses the correlation coefficient to reflect the correlation between indexes. The larger the standard deviation is, the greater the differences in the values of each evaluation object under the same index and the greater the weight. The larger the correlation coefficient is, the smaller the conflict between indicators and the smaller the weight. The calculation steps of determining weights based on the CRITIC method are as follows:
The first step is to calculate the standard deviation of each evaluation index σj according to Eq. (8):
where xj¯ is the average value of index j.The second step is to calculate the correlation coefficient between each index rij according to Eq. (9):
Step 3 is to calculate the weight of each primary index, as shown in Eqs. (10)-(11), where the larger the value of Cj is, the greater the role of evaluation index j in the whole evaluation index system. Thus, more weight should be assigned to it:
Based on the improved G1 method and the CRITIC method described above, the weights between the secondary indicators within the primary indicators and the weights between different primary indicators, respectively, can be obtained. Additionally, the final weights can be calculated by multiplying them. Finally, the weights of all indicators can be obtained, as shown in Table 2, in which the numerical values after the names of the secondary indicators represent the order relation.
Weight of each level.
To calculate the performance score of collaborative innovation centers, this study adopts the TOPSIS method, which is suitable for dealing with multicriteria decision-making problems (Pena, Napoles & Salgueiro, 2021). This method judges the merits of the evaluation object by calculating the distance between it and the positive ideal solution and the negative ideal solution. The closer the index value is to the positive ideal solution, the higher the score. The method is characterized by consistency and simple calculation.
The main calculation steps are as follows:
The first step is to build evaluation matrix D according to Eq. (12):
The second step is to multiply decision matrix D above by the weight of each indicator ωj obtained above to obtain weighted decision matrix D2 as Eqs. (13)-(14):
Step 3 is to obtain the positive and negative ideal solutions through the weighted decision matrix, that is, to obtain the maximum and minimum values of each column of the matrix, as shown in Eqs. (15)-(16), where tj+ is the optimal solution of index j and tj− is the worst solution of index j:
Step 4 is to calculate the Euclidean distance between the index and the optimal solution and the worst solution as according to Eqs. (17)-(18):
Step 5 is to calculate the final score CCi of each collaborative innovation center by synthesizing the distance from the best point and the distance from the worst point according to Eq. (19):
Step 6 is to compare the scores of collaborative innovation centers and rank them. The ratings are made based on the final ranking results. The rating table is shown in Table 3.
Because the scores calculated by the TOPSIS method are small, they do not intuitively reflect the performance of collaborative innovation centers. To better reflect their performance, this paper maps all the calculated scores within the interval of [3, 10] based on min-max normalization.
Performance evaluation results analysis and displayIn this section, we present and discuss the obtained performance results. First, in Section 4.1, we present the main issues regarding system implementation. Then, in Section 4.2, we present and analyze the obtained results. Subsequently, in Section 4.3, we compare the results obtained by the proposed methodology to those computed using a reference ranking method.
System implementationOn the basis of constructing the performance evaluation method, based on Python and MongoDB, the corresponding performance evaluation system for collaborative innovation in universities is implemented. The functions of the system correspond to the main tasks in the data life cycle, and they mainly include data input, diversified data query, outlier analysis, comprehensive evaluation, performance data display and comparison of the performance evaluation results. There are different data functions available to different participants. Here, due to space limitations, we focus on the function of the comprehensive evaluation method. This function includes not only the evaluation method proposed above but also three other classic comprehensive evaluation methods, namely, the ANP, EW method and G1-EW method. Through the performance data display function, the system can show the basic situation of and changes in various indicator data for each collaborative innovation center from 2015 to 2019. The performance evaluation result comparison function provides users with the evaluation results of different subjects and the comparison results of different evaluation methods. The display interface of the performance evaluation system for collaborative innovation in universities is shown in Fig. 2 below.
Analysis of the evaluation resultsAll indicators are weighted by the improved G1-CRITIC method described above, and the final score is obtained by the TOPSIS method and then classified and sorted by year. Each university collaborative innovation center is rated based on the score table. Taking the university collaborative innovation centers at Hohai University as examples, Fig. 3 below shows the evaluation results of the four collaborative innovation centers at Hohai University in 2018. In 2018, the four centers received two A ratings, one B rating and one D rating. Among them, the development of center 2 is far higher than that of the other three centers and ranks among the best in the province. However, the development of center 1 is not satisfactory, meaning that the center needs more investment and support from this university.
This paper analyzes in detail all aspects of the development of collaborative innovation centers by calculating the scores of eight different types of subitems, namely, the composition of capital investment, newly trained and introduced talent, the composition of current employees, international exchanges and cooperation, major awards, the paper output, the patent output, and service and contribution to society. The scores of the performance subitems of the collaborative innovation centers at Hohai University in 2018 are shown in Fig. 4 below. From the perspective of the individual scores, Hohai University has done well in terms of international exchanges, major awards and the paper output. However, there is still much room for improvement in terms of new talent training, the patent output and service and contribution to society.
Results analysis and comparisonTo further verify the credibility of the experimental results, the results of the comprehensive evaluation methods mentioned above are compared with those based on an objective weighting method, a subjective weighting method and a combined weighting method. Here, the EW method is selected as the objective weighting method, the ANP method is used as the subjective weighting method, the G1-EW method is used as the combined weighting method, and the TOPSIS method is used for the final scores and ratings. Fig. 5 shows the scores of the collaborative innovation centers at Hohai University in 2019 under different comprehensive evaluation methods.
Compared with the scores obtained by some typical comprehensive evaluation methods, the final score of the improved G1-CRITIC method does not show a very large difference, and all of the scores fluctuate within a certain range. However, based on the final rating results, there is a large gap between the comprehensive evaluation method based on the EW method and the other three methods. These results are a sample rating for a single university collaborative innovation center. To further illustrate the overall performance trend of the evaluation system, the results of all collaborative innovation centers in 2019 are listed next. Fig. 6 shows the scores of 73 different collaborative innovation centers in universities in Jiangsu Province in 2019.
Fig. 6 shows that the comprehensive evaluation method adopted in this paper has basically the same trend as the other comprehensive evaluation methods. However, the comprehensive evaluation method based on the EW method has some points of deviation because its weighting process is completely based on numerical changes and has difficulty reflecting subjective factors. A single objective weighting method has difficulty obtaining good results when there are a large number of related indicators, and it needs to be controlled by subjective factors. However, subjective weighting methods such as the ANP require considerable time and energy when weighting, the evaluation efficiency is low, and the index weight is relatively fixed, meaning that such methods cannot cope with the changes in real-time data. Based on the changes in objective data, the proposed weighting method can add certain subjective factors to reflect the correlation between data with accuracy and efficiency. Additionally, the method can objectively and effectively evaluate the performance of collaborative innovation centers.
There are four different types of collaborative innovation centers in colleges and universities: those dedicated to industry, regional development, cutting-edge science and cultural heritage. By the end of 2019, there were thirty-six industry-oriented collaborative innovation centers, twenty-eight collaborative innovation centers for regional development, three collaborative innovation centers for cutting-edge science and seven collaborative innovation centers for cultural heritage. Among them, the probabilities of the four types of collaborative innovation centers reaching A and B ratings from 2015 to 2019 are shown in Fig. 7.
As shown in Fig. 6, 55.1% and 51.8% of collaborative innovation centers for industry and regional development in Jiangsu universities have A and B ratings, respectively. In contrast, only 34.3% and 20% of centers for cultural heritage and cutting-edge science have A and B ratings, respectively. Indeed, collaborative innovation centers for cultural heritage and cutting-edge science are far behind the other two types of collaborative innovation centers in terms of the number of collaborative innovation centers, capital investment and the collaborative output of innovation. Therefore, there is not enough competitive advantage in the evaluation system. For the coordinated development of collaborative innovation centers, it is necessary to pay attention to the construction of this type of platform and to increase investment and policy guidance.
In terms of knowledge, this study draws the following key conclusions. The overall level of construction of collaborative innovation centers in Jiangsu Province is relatively high, but the overall development is unbalanced, and the level of performance of different types of collaborative innovation centers is significantly different. As the data continue to be updated, multiple party participants can use the diversified functions of the performance evaluation system to continuously obtain the development status of collaborative innovation in universities from various perspectives. Of course, the results of comparison and clustering among collaborative innovation centers in Jiangsu Province can also be obtained.
ConclusionsInnovation has become the key for organizations to build sustainable competitive advantage. In this paper, a performance evaluation method based on an improved G1-CRITIC comprehensive evaluation model for collaborative innovation is proposed and discussed. Then, the corresponding performance evaluation system for university collaborative innovation is implemented. At the same time, through comparison with other comprehensive evaluation methods, the effectiveness and usability of this method are verified. In terms of knowledge, we can not only obtain the general situation of collaborative innovation centers in Jiangsu Province but also compare the differences between different collaborative innovation centers.
The curation of data on collaborative innovation in universities is the active and on-going management of data. Future research will focus on using and integrating machine learning and knowledge graph methods to analyze collaborative innovation data. By further analyzing and comparing the differences between different methods, the corresponding evaluation system will be customized for each subject, which will facilitate each subject in carrying out the corresponding work.
The paper was supported by the Jiangsu Social Science Fund Project “Jiangsu University Collaborative Innovation Mechanism and Efficiency Research” (No. KJ20210178); the Jiangsu Province Innovation Support Program Soft Science Research Project "Construction and Research of Jiangsu University Science and Technology Personnel Evaluation System" (BR2022009); and the Natural Science Research Project of Jiangsu Universities "Monitoring and Research on the Construction Procedure of Collaborative Innovation Center in Jiangsu Universities" (20KJB630008).