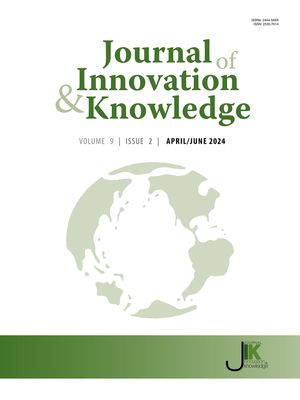
Edited by: Brij B. Gupta, Kwok Tai Chui
More infoWith efficient and effective access to information technology, exports could be the best solution for businesses to tackle climate change through “learning by exporting” for sustainable development. With this context as reference, this study uses the directional distance function and the Malmquist–Luenberger Productivity Index (MLPI) to analyze the “green” total factor productivity (GTFP) growth of exporting firms in India by comparing them with non-exporting firms from 2012 to 2020. The finding reveals that, on average, GTFP growth is higher for the exporting firms (7.9%) as compared to non-exporting firms (-0.7%)—the “learning by exporting” effect exists in India, and exports could help Indian firms tackle climate change. Moreover, the decomposition of the MLPI shows that technical change (TC) is the main driver of GTFP in both exporting and non-exporting groups—IT or technological innovation leads to an increase in environmental productivity. Furthermore, the Tobit model is used to analyze the main drivers of TC that promote the GTFP in both groups. The results show that the product innovation (0.61%) has exerted a remarkable positive effect on promoting the GTFP of exporting firms and the human capital quality (0.57%) in non-exporting firms. Based on these findings, we propose that Indian policymakers “prudently” implement more field-oriented or performance-oriented policies for firms through promotional policies, such as tax incentives, while also encouraging the adoption of innovative technology for more effective mitigation of climate change for sustainable development.
Climate change—caused by greenhouse gas emissions, primarily carbon dioxide (CO2)—has become a rising source of concern in recent years, posing serious challenges and risks to the environment, as well as humanity and its way of life (Kumar et al., 2021). To address this issue, numerous experts and policymakers worldwide have shared their perspectives and have proposed various mechanisms and ways to mitigate climate change for sustainable development. Exporting could be the simplest approach to mitigating climate change by promoting technological innovation because global business is becoming more competitive, and environmental protection has become the new norm. Thus, exporting firms better understand how to mitigate climate change (Xie & Li, 2018). Consequently, firms will be more willing to invest in technological innovation to grow steadily and achieve long-term sustainability. However, there are two different schools of thought about exporting: the “learning by exporting” effect or the “pollution heaven” effect. In this study, “learning by exporting” refers to the mechanism whereby a firm's productivity performance improves after entering the export business (De, 2013). This is because entry into the export business improves the agility for firms to adopt new norms, such as climate change, on best managerial practices, technological innovation, and exposure to much more severe global competition (Clerides et al., 1998). The “pollution heaven” effect states that exports may damage the production country's environmental quality, while the “learning by exporting” effect may not always exist in some emerging exporting countries (Sarkodie & Strezov, 2019; Solarin et al., 2017). Thus far, there has been limited study concerning India, which, as an emerging exporting country, also suffers from these conflicts over exports—the “learning by exporting” effect or the “pollution heaven” effect.
India's exports of goods and services were worth $ 39.069 billion at the time of its joining the World Trade Organization (WTO) in 1995. It increased to $529.016 billion in 2019, accounting for 18.429% of India's GDP (The World Bank, 2022a, 2022b). Fig. 1 shows that India's exports increased significantly between 1995 and 2019, owing to strong support from the Indian government. Simultaneously, India's CO2 emissions also increased significantly over the same period (The World Bank, 2022c), mostly due to rapid growth in manufacturing output (Barrows & Ollivier, 2021). Thus, there might be a direct relationship between export growth and CO2 emissions in India; when exports rise, so do CO2 emissions. With its massive increase in exports and CO2 emissions, India provides an excellent opportunity to investigate the contradicting views about the exports of developing countries on the new norm of climate change mitigation under the WTO.
Since 2009, India has been the world's third-largest contributor to CO2 emissions, after China and the United States (The World Bank, 2022d). In response, the Indian government has encouraged efforts to improve energy efficiency and reduce carbon emissions through many initiatives. On October 2, 2016, India ratified the Paris Agreement with its first nationally determined contributions (NDC). Based on the NDCs, the Indian government has pledged to reduce the emission intensity of GDP by 33–35% by 2030, compared to 2005 levels, through technology transfer and low-cost international funding, particularly from the Green Climate Fund (Climate Action Tracker, 2021). However, it is unclear whether Indian exporting firms have reduced CO2 emissions voluntarily, considering India's support for the Paris Agreement, and whether the country's commitment to climate change mitigation can set it on the path toward sustainable low-carbon development in the decade ahead.
Based on the preceding discussion, this study addresses the following research questions: Are exports beneficial in reducing CO2 emissions in India? (Or does “learning by exporting” affect India?) Does India's commitment to climate change mitigation by reducing CO2 emissions follow the “learning by exporting” effect or the “pollution heaven” effect for exporting firms? If exports promote the environmentally friendly performance of the economy, what are the main drivers of productivity growth? This study aims to answer all these research questions by examining the green productivity growth of Indian exporting firms and proposing specific policy recommendations for a “greener” economic transformation. Green productivity is a strategy for improving both productivity and environmental performance to achieve sustainable development using a set of managerial tools and technologies that reduce the environmental impact of a firm's activities to encourage technological innovation and a continuous cycle of productivity gains (Global Development Research Center, 2022). In this context, this study assesses the NDC policy's impact on the GTFP growth of India's exporting firms by comparing them with non-exporting firms and investigate the main drivers of GTFP growth.
This study contributes to the literature in four ways. First, we use a directional distance function (DDF) and a Malmquist–Luenberger Productivity Index (MLPI) to estimate the dynamic changes in the GTFP of India's exporting and non-exporting firms using a unique dataset from 2012 to 2020. To the best of our knowledge, no studies in India have assessed the GTFP of exporting firms (compared with non-exporting firms) based on emission reduction policies, considering CO2 emissions as an undesirable output. Further, we could not find any studies dealing with exports as a mechanism for reducing CO2 emissions in the Indian context through the “learning by exporting” effect. Therefore, this is the first study to compare the GTFPs of India's exporting firms to those of non-exporting firms. This study seeks to bridge the research gap by proposing useful and strong policy alternatives. Second, we decompose the GTFP into two indices—efficiency change (EC) and technical change (TC)—to identify the main drivers of GTFP growth. Third, this study uses an econometric model to estimate the factors that promote the GTFP. Finally, we suggest some policies based on our findings.
The rest of the paper is organized as follows. Section 2 includes a brief review of the related literature, and Section 3 describes the methodology and data description. Further, Section 4 presents the results and discussions, and Section 5 concludes the study by providing some policy implications.
Literature review and contextual settingTheories about exports, CO2 emissions, and emission reduction policiesDuring the last decade, discussions and research on climate change due to CO2 emissions have attracted considerable attention (Hinge et al., 2021; Jha et al., 2021). Exporting could be the easiest way to increase firms’ GTFP and reduce CO2 emissions by promoting technological innovation. This is because exporting firms compete with global firms and must learn how to mitigate climate change for sustainable development. However, recent studies on the impact of trade (exports) on firm performance have found it arduous to establish conclusive evidence. For example, there are two schools of thought regarding exports. The first school argues that exports force the host country to develop its technology to meet the rising customer demand for products, thereby improving the production country's environmental quality. That is the “learning by exporting” effect (Damijan & Kostevc, 2015; Liu & Lin, 2018). Forslid et al. (2018) estimated the relationship between export trade and CO2 emissions using firm-level data from Sweden. Empirical evidence suggests that exports help reduce CO2 emissions. Fernandes and Isgut (2015) thoroughly examine the “learning by exporting” effect hypothesis for Colombian manufacturing plants and find substantial evidence, using different samples, econometric methods, and modeling approaches. They found evidence of a lack of a “learning by exporting” effect for exporters that drop from export markets. Another school of thought argues that the emissions generated in the production of goods and services to meet export demand are added to the producing country's emissions account (Cui et al., 2011). In such cases, the environmental consequences of good production harm the ecological system of the exporting country (production site) rather than that of the importing country (consumption site). This is the “pollution heaven” effect (Caro et al., 2017). Stretesky and Lynch (2009) examined the impact of export trade on CO2 emissions using panel data from 169 countries and found that overall, export trade will increase CO2 emissions.
Similar to export theories, some studies argue that emission reduction policies impact exporting firms positively; others contend that regulatory policies have little or no impact on exporting firms for sustainable growth. Iwanow and Kirkpatrick (2007) discovered that environmental regulations impact exports considerably. According to the author's findings, while trade facilitation can help enhance export performance, improvements in the regulatory environment and basic transportation and communications infrastructure are equally crucial, if not more so, in promoting export growth. Pavcnik (2002) examined the impact of tariff reductions on export performance in Chile using firm-level data and discovered that tariff reductions encourage firms with lower productivity to exit the market. However, Crespi and Costantini (2007) revealed that the introduction of more rigorous environmental rules has long been considered potentially deleterious to the national industry's productivity and competitiveness because it leads to increased costs for firms. Copeland & Taylor (2013) suggested that governments tend to reduce manufacturers’ costs through more lenient environmental restrictions, thus boosting their international competitiveness.
Based on the preceding discussions, theories on exporting have not achieved consensus in research theoretically and empirically. The same can be noted about regulatory policies, with there being a mixed view on their impact on exporting firms. Furthermore, previous studies have shown that the relationship between exports and CO2 emissions has received considerable attention in academia. The DDF and MLPI have been used in prior studies to estimate the GTFP of exporting firms in their host country at the firm level (Barrows & Ollivier, 2021; Rath, 2018), and the same method was applied in this study.
GTFPAccording to Tuttle and Heap (2008), the Asian Productivity Organization was the first to introduce the concept of green productivity, following the Rio Earth Summit in 1992. The “green productivity” of an industry can be interpreted in terms of the level at which environmentally friendly technologies are used and the cost of adopting them.
As per new classical theories, the degree of contribution of technological innovation determines an economy's viability (measured by total factor productivity) (Tian & Lin, 2017). For example, total factor productivity can be calculated using a regression analysis of production functions based on Solow's Growth Model (1956). However, traditional methods do not account for pollutants or by-products, such as CO2 emissions, regarding environmental and economic studies. Several approaches have been proposed to address this problem. For example, the DDF from the original data envelopment analysis (DEA) model is a measure of efficiency that has been used in many comparable studies based on the works of Farrell (1957) and Charnes et al. (1978). The DDF considers input parameters and desirable and undesirable outputs such as CO2 and other pollutant emissions in production technologies. Färe et al. (1994) proposed the Malmquist index (MI) to measure dynamic productivity change, which has since become widely used in productivity measurement and analysis. MI is based on an efficiency measure that can be determined using parametric and non-parametric methods (Tian & Lin, 2017). Chung et al. (1997) proposed and developed the MLPI based on the DDF and MI to evaluate environmentally sensitive productivity growth (also known as real or GTFP) using time series data while adjusting for undesirable outputs in the ecological environment.
Since then, many studies on efficiency and productivity measurement have been conducted using the MLPI in various fields; thus, the MLPI is a good indicator of the GTFP (Chen & Golley, 2014; Li & Lin, 2016). To determine the main drivers of productivity growth, the MLPI can be further decomposed into two components: EC and TC. EC (also known as the catching-up effect) captures how close observation is to the production frontier, whereas TC (also known as the technological innovation effect) measures shifts in the production frontier, which can be viewed as an indicator of the impact of innovation.
The methodology described above has been widely used to measure efficiency and productivity change in the presence of undesirable outputs at the national, regional, and firm levels (Barrows & Ollivier, 2021; Lin et al., 2013). However, there has been little research on the full comparison of exporting and non-exporting firms to assess the GTFP performance of exporting firms based on emission reduction policies in India—the second most emerging economy after China and the third in terms of CO2 emissions. Therefore, to assess the feasibility of Indian NDCs under the Paris Agreement, this study employs the DDF and MLPI to estimate the GTFP performance of exporting firms in comparison with non-exporting firms in India.
Methodologies and dataModeling technology with desirable and undesirable outputBased on the traditional approach to production, the model begins with three basic inputs: capital, employee, and energy consumption. These inputs generate a desirable output of sales turnover and undesirable output of CO2 emissions (Choi et al., 2020; Lee & Choi, 2019). The same indicators were used in this study to assess the efficiency of India's manufacturing firms between exporting and non-exporting.
Assume that we have N manufacturing firms and that each firm produces a vector of desirable outputs y∈R+M and undesirable outputsb∈R+O) at time t,t=1,2,3,…,T, using a vector of inputs x∈R+L. Subsequently, using T(x) as the feasible output set for the given input vector, we describe the technology in Eq. (1) using its output sets.
Here, T is assumed to satisfy all standard production technology axioms (Färe & Grosskopf, 2005)—inactivity is always possible, and finite quantities of a given input can only produce finite quantities of output. According to Färe et al. (2007), desirable outputs must be freely disposable, undesirable outputs must be weakly disposable, and the by-product axiom of T must be satisfied.
P.1.The free disposability of desirable outputs (y, b)∈ P(x) and y′≤yimply(y′,b)∈P(x).
P.2.Weak disposability of undesirable outputs: (y, b)∈ T(x), and 0≤θ≤1imply(θy,θb)∈P(x).
P.3.By-product axiom: (y, b)∈P(x) and b=0 then y=0.
According to the free disposability assumption (P.1), reducing the undesirable output is feasible only if the desirable output is simultaneously reduced, given a fixed level of inputs. According to the weak disposability assumption (P.2), reducing undesirable outputs (e.g., CO2 emissions) is expensive. As per the by-product axiom assumption (P.3), desirable outputs are “null-joint” with undesirable outputs if the only way to produce no undesirable output is to generate zero desirable outputs. Alternatively, if a desirable output is produced in a positive amount, some undesirable output must also be produced.
Directional distance functionThe technological set can be built using the DDF based on the given production assumptions of free disposability, weak disposability, and by-product axiom. The output-oriented DDF used to measure the efficiency of the observations is defined in Eq. (2) (Chung et al., 1997).
where g=(gy, −gb)∈R+M×R+Orepresents the direction of desirable and undesirable outputs. The benefits of this function include the abilities to make non-proportional changes to outputs and inputs and expand one output while contracting another, which is extremely useful when studying input/input choices. The output DDF is shown in Fig. 2. The technology is represented by the output set T(x)to which the output vector of point (y, b) belongs, where y and b denote desirable and undesirable outputs, respectively. The DDF simultaneously credits increases in desirable output and reduces undesirable output in the estimation of a production frontier by taking the same output vector from A to B—in the direction of g=(gy,−gb) (Lin et al., 2013). This means that if the firm moves from A to B, it would be judged as efficient based on the DDF. The technology bounded by the line segments OR, RS, and ST represents the production frontier. The technology bounded by line segments OQ, QR, RS, and ST represents the free disposability, where it could expand y and contract b to be at point C on the boundary.The DDF for observation N in Eq. (3) can take the following linear programming form (Färe et al., 2001):
Malmquist–Luenberger Productivity IndexThe output-oriented DDF considers output quantities to be proportionally expanded without altering the input quantities used. The Malmquist approach is most commonly used for output comparisons (Wadud & Paul, 2006). Hence, this study adopts an output-oriented approach for computing GTFP (Rath, 2018). The output-oriented MLPI can be calculated further based on the DDF to check how efficiency improves over time in Eq. (4) (Tian & Lin, 2017).
The MLPI measure indicates productivity improvements if its values are greater than 1 and decreases in productivity if the values are less than 1 (Chung et al., 1997). The MLPI can then be decomposed into two mutually exclusive and exhaustive parts—one measuring EC and the other measuring TC—to examine the main drivers that contribute to the dynamic change in the GTFP growth in Eq. (5).
The “catching-up” effect is represented by the EC index in Eq. (5), which focuses on the evolution of the gap between a production unit and the frontier between periods t and t + 1, indicating changes in manufacturing firms’ GTFP—EC represents the change in the distance between a specific firm and an effective frontier. Between the two periods, TC reflects the advancement of the most advanced technology available, as reflected by the frontier of the production process; this is referred to as a “technological innovation effect.” If TC>1, it allows for more desirable and less undesirable outcomes; if TC=(<)1, it indicates that production technology is either unchanged or deteriorating. We can obtain the MLPI for Indian manufacturing firms (exporting and non-exporting) using the DDF function, which measures GTFP (or green productivity growth). MLPI decomposition is then performed to determine how the efficiency and technology change over time. Furthermore, these tools can be used to determine which of the two components is the main driver of the GTFP growth in India's exporting and non-exporting firms.
Data descriptionsTo examine the GTFP performance of Indian manufacturing firms (exporting and non-exporting), data were collected from 52 manufacturing firms in India (28 exporting and 24 non-exporting firms) during 2012–2020, considering the data's consistency and availability. The following sectors were chosen for this study: oil, gas, and energy; mining and metals; pharmaceuticals and chemicals; iron and steel; cotton and textiles; agro-food; others (Fig. 3). As previously stated, the input variables are capital, employees, and energy. Sales turnover (desirable) and CO2 emissions (undesirable) are output variables. All variable data were gathered from each Indian manufacturing firm's annual report, which is available on their respective websites (Choi et al., 2020). Firms provide detailed accounts of input and output variables in their annual reports. For capital input, we extracted fixed asset data from each firm's annual report from 2012 to 2020. In terms of employee input, we gathered the number of employees per head of the firm. For energy input and CO2 emission output, we collected data on power and fuel consumption rates at the macro level, from exporting and non-exporting firms, following the International Panel on Climate Change guidelines (Choi et al., 2020). We gathered the income generated by the firm's operations to calculate the sales turnover output.
As this study aims to compare the performance of exporting and non-exporting firms in India, we have categorized the groups as such. Goods produced in one country and sold in another country or a service provided in one country for a national or resident of another country are offered by exporting firms. Non-exporting firms are not linked to exports. Each group of firms may respond differently to the government's emission reduction policies on manufacturing firms and thus may result in more precise implications and customized suggestions in this study. Therefore, it is critical to examine these two types of firms and evaluate their performance in terms of sustainable development. On this basis, we compare the performance of exporting and non-exporting firms to assess their GTFP and whether there is a difference in productivity efficiency models between these groups. The descriptive statistics for the input and output variables are shown in Table 1.
Descriptive statistics of the inputs and outputs, 2012–2020.
Table 2 displays the correlation matrix of the input and output variables for the exporting and non-exporting firms from 2012 to 2020. The results demonstrated a favorable correlation between the input and output variables—the output variables increase or decrease depending on the input used during the production process. As capital and employees are representative variables for explaining production, they are positively related to sales turnover. In particular, capital and sales turnover (.904) demonstrate a significant relationship. Further, there is a significant relationship between capital and energy consumption (.640)—when a firm purchases and upgrades energy-efficient equipment, it also affects its energy consumption (Hu, 2012). While both sales turnover and CO2 emissions have a positive association with energy, the energy and CO2 emissions link (0.910) is highly significant. The findings of this study validate the importance of analyzing data from an environmental perspective.
Empirical results and discussionsMLPITable 3 presents the empirical results of the average MLPI and its decomposition for Indian exporting and non-exporting firms between 2012 and 2020. Owing to the confidentiality of firms, we used the firm ids (acronyms) in Table 3. The results demonstrate an increase in the GTFP performance of exporting firms between 2012 and 2020, whereas non-exporting firms exhibit a decline.
Average MLPI and its decomposition in exporting and non-exporting firms.
According to the MLPI estimation, the GTFP performance of exporting firms increased by approximately 7.9% on average—exporting firms made progress in the GTFP during the study period. The non-exporting firms show a 0.7% decrease in GTFP performance. At the firm level, 20 exporting firms experienced an increase in productivity performance, whereas 8 firms experienced a decrease in value. Among non-exporting firms, 6 firms show increased productivity performance, while the remaining 18 firms show a decline. This finding shows that exporting firms outperformed non-exporting firms in terms of adopting the environmental regulatory policy of NDCs under the Paris Agreement during the study period.
As previously stated, we decomposed the MLPI into two indices—EC and TC—to examine the main drivers of GTFP growth. The average EC index of the GTFP performance for exports is 1.002 under the MLPI framework, indicating a strong catching-up effect. In the case of non-exporting firms, the catching-up effect decreases by 0.9% annually—the EC plays a negative role in MLPI promotion. Further, 16 exporting and 5 non-exporting firms have an EC index greater than unity—these firms are moving toward the environmental technology frontier; consequently, the EC is catching up more quickly with exporting firms. Meanwhile, the average TC index for both groups was greater than unity, with an annual increase in the technological innovation effect of 7.7% (exporting) and 0.2% (non-exporting)—TC promotes green productivity growth. Additionally, 21 exporting firms demonstrated technological progress, whereas 17 non-exporting firms had a value greater than unity. Furthermore, the average TC values of exporting and non-exporting firms were higher than EC values—the TC index has a greater influence on the favorable effect of the GTFP in Indian manufacturing firms (Table 3). Consequently, we can say that GTFP growth in Indian manufacturing firms is driven mainly by TC (or technological innovation) in environmental efficiency.
Table 3 shows that exporting firms exhibit an improvement in GTFP, while non-exporting firms show a slight deterioration. To examine whether there is a significant difference in the MLPI between exporting and non-exporting firms, this study used the Wilcoxon-Mann-Whitney rank sum test, with the results shown in Table 4. At the 95% confidence level, the results of MLPI, EC, and TC reject the null hypothesis—there are statistically significant group differences between exporting and non-exporting firms in terms of productivity performance and its decomposition in Indian manufacturing firms. The distribution patterns of these indices differ between exporting and non-exporting firms, as shown in the kernel density plot in Fig. 4. This difference in groups prompted us to investigate the dynamic trends in GTFP performance, breaking it down between exporting and non-exporting firms from 2012 to 2020. This trend could provide useful information for Indian policymakers to negotiate with both groups in terms of carbon emission reduction targets based on their performance because the MLPI could provide information for both the catching-up and technological innovation effects considering the group heterogeneities.
The significance level is 0.05.
Fig. 5 and Appendix A show the GTFP performance trends for exporting and non-exporting firms over the period 2012–2020 based on the MLPI calculation. On average, the MLPI of exporting firms shows an increasing trend—the GTFP of Indian exporting firms improved during the study period, suggesting that exports could be beneficial in mitigating CO2 emissions for Indian firms through the “learning by exporting” effect. The decrease in 2015–2016 could be mainly due to the global demand slowdown and the sharp drop in export commodity prices and exchange rates, both of which heavily affected Indian export firms (The Economic Times, 2016). Meanwhile, the performance was observed more clearly after 2015–2016, which could be due to India's first NDC commitment to mitigating climate change. As previously reported, in 2016, the Indian government ratified a clear and strong reduction target for CO2 emissions under the Paris Agreement, known as NDC (Choi et al., 2020). Consequently, all firms emitting the most CO2 have been under pressure to reduce CO2 emissions to meet the Indian government's 2030 objective. Therefore, performance was observed more clearly after 2015–2016. The unprecedented decrease in the volume of exports in 2019–2020 could be due to the global economic crisis following the outbreak of the COVID-19 pandemic, with exports decreasing due to reduced demand for textiles, clothing, and other goods in global business (The Hindu, 2020). Additionally, some large Indian exporting firms, such as Grasim Industries, Tata Motors, and ITC, have temporarily suspended or reduced operations at several manufacturing facilities and factories across the country, leading to a decrease in their sales turnover. A reduction in sales turnover may lead to decreased productivity in a firm. Despite a slowdown in productivity performance in 2019–2020, India's first NDC is following the “learning by exporting” effect for exporting firms. For non-exporting firms, the GTFP performance for the 2012–2020 period shows no change in growth, which is near unity, going above and below during the sample period. We suggest that Indian policymakers should be aware of this phenomenon and take suitable measures to decrease the productivity disparities between exporting and non-exporting firms.
Fig. 6 depicts the trends in the MLPI EC index for exporting and non-exporting firms. For exporting firms, the EC index shows an increasing trend (M-shaped). For example, the EC index had a value less than unity for the 2012–2013, 2016–2017, and 2018–2019 periods, indicating that catching-up performance decreased during those periods and vice-versa for other periods. Indian policymakers need to investigate this to determine why there is an M-shaped pattern in the growth rate efficiency of exporting firms in India to customize the existing policy for easy adoption by exporting firms. For non-exporting firms, the EC index for 2012–2014 shows a value less than unity, indicating that catching-up performance decreased during these years. However, for the 2014–2020 period, the EC index remained near unity, occasionally rising or falling, indicating that the catching-up performance remained unchanged. On average, the export group has a higher EC index. This suggests that during the study period, India's exporting firms improved their efficiency as a result of the best-practice frontier's catching-up effect. Therefore, Indian policymakers should not overlook non-exporting firms in terms of emission reduction targets and should treat both groups equally for sustainable development by establishing more rigorous, predictable policies for both exporting and non-exporting firms in India.
Fig. 7 depicts trends in the TC index of the MLPI for exporting and non-exporting firms. Overall, the exporting group has a higher TC index than the non-exporting group—greater technological innovation performance and rapid equipment and technology upgrades have benefited the exporting group. However, exporting firms experienced a sharp drop in the TC index from 2019 to 2020. As previously stated, the COVID-19 pandemic may have influenced Indian exporting firms initially, resulting in a significant drop in innovative activities with little or no inputs (humans and machinery) invested, leading to a decline in technological innovation in 2019–2020.
In summary, the non-exporting group lacks both a catching-up effect and a technological innovation effect; therefore, there is a missing link in the role of the central government of India for the non-exporting group, which is the backbone of the Indian economy. The Indian government should encourage non-exporting groups to learn from exporting groups in terms of both the catching-up effect and technological innovation effect to improve their own GTFP performance for sustainability.
Main drivers of technological changeThe previous section briefly analyzed GTFP growth and its decomposition by comparing exporting and non-exporting firms from the period 2012–2020. The results show that the exporting group performs better in terms of GTFP growth and that TC is the main driver of GTFP growth in Indian manufacturing firms, based on the MLPI estimate. However, the question as to what the main drivers of TC that promote GTFP growth in manufacturing firms are remained unanswered in the previous subsection. To address this issue, we specify an econometric model to identify the main drivers of TC that promote the GTFP growth. Examining the main drivers of GTFP growth is an important step toward understanding the significant factors that affect the productivity of manufacturing firms in the long run for sustainable development (Rath, 2018). It will also help policymakers and firms understand the action to undertake to facilitate technological progress and mitigate climate change.
Technological innovation has been characterized through variable measures, such as research and development (R&D), human capital quality (HCQ), patents, products, and processes by existing studies (Rasiah et al., 2010; Rodríguez & Rodríguez, 2005). R&D expenditure is widely believed to play a key role in enhancing firm performance. Aw et al. (2011) stated that spending on R&D enhances a firm's stock of knowledge, leading to better innovation, which can increase output due to increased productivity. Thus, R&D is considered one of the key drivers of technological innovation. According to Zhang et al. (2019), HCQ has a significant impact on productivity; the higher the HCQ, the greater the productivity. Human capital can improve workers’ skills and the absorption of advanced technology, resulting in “technical spillovers,” which increase the GTFP. Thus, HCQ is an important driving force in promoting manufacturing firm quality. Over time, periods with a high number of influential patents are followed by those with a high rate of productivity growth. Patents have the advantage of being the result of an innovation process (Amable & Verspagen, 1995). Consequently, patents may serve as indicators of specific types of technological innovation. As important as other TCs, new product development also seems to have been conducted more heavily by manufacturing firms. Designing new products in response to new market demands is essential for product diversification and productivity (Kirim, 1990). Thus, there appears to be a relationship between manufacturing firms and this type of TC activity. Process innovation involves the implementation of a new or significantly improved production or delivery method. This includes significant changes in techniques, equipment, and software. However, if the introduction of process innovations stops, innovation appears to be associated with an end to productivity growth in the following years (Huergo & Jaumandreu, 2004). We collected all explanatory variables from the annual reports of each firm for 2020 to deploy an econometric model in this study. We chose the MLPI scores as the dependent variable (Table 3). Table 5 displays the descriptive statistics for the explanatory variables for both exporting and non-exporting firms.
Descriptive statistics for variables in exporting and non-exporting firms, 2020.
To determine the best model specification, two models were tested: (a) an ordinary least squares (OLS) model and (b) a Tobit model. The OLS model is used to identify the variables that best characterize TC (technological innovation) between exporting and non-exporting firms. In this case, a truncated estimation procedure was used as the dependent variable (MLPI score) and was observed only if it was greater than zero (Song et al., 2020). In summary, the OLS regression model assumes the following form (Nassimbeni, 2001):
where the dependent variable is GTFP growth, and the independent variables are TC and EC. TC covers a wide range of innovative areas, including R&D, HCQ, patents, products, and processes. However, the Tobit model enables all the available information from the explanatory variables to be used but includes both the decision to identify the variables that best characterize TC (technological innovation) and whether the variables affecting the GTFP are different in both firms in a single model. Tobit regression analysis was chosen in preference to the more common least-square method because the dependent variable has a censored distribution (1 if the MLPI average value is more than unity and 0 if the MLPI average value is less than unity). The Tobit regression model has been widely used in research on energy efficiency (Liu & Lin, 2018) and environmental efficiency (Zhang et al., 2016), which provides an important reference for this study. Additionally, the Tobit regression model can avoid bias and inconsistency when used to estimate unknown parameters. Therefore, based on the Tobit regression approach, we define the following econometric model (Ayaz et al., 2010).yit = explained variable; xit = explanatory variable; βT = vector of the regression coefficient of the explanatory variable; εit = the stochastic error assumed to follow the distribution of N (0,σ2).To assess the main driver of GTFP, the Tobit model is defined in Eq. (8) (Kuang et al., 2020):
where GTFPis the dependent variable, iandt represent the ith firm and year, βi is the coefficient, TC (R&D, skill, patents, product, and process) and EC are the explanatory variables, and eit is a stochastic error.The results of the statistical analysis are reported in Table 6, which shows the coefficients and significance of each independent variable of the two models by comparing exporting and non-exporting firms. Table 6 shows that the Tobit estimates follow a distinct pattern from the OLS estimates in terms of the coefficient and significance level. For example, in the Tobit model, R & D has a strong negative influence on the GTFP of exporting firms with a 1% significance level, whereas OLS has a negative effect but with a 5% significance level. A similar phenomenon may be observed for HCQ for exporting firms with a different significance level, where the Tobit model outperforms the OLS model. Additionally, the Tobit model's coefficient is larger than that of OLS for all the explanatory factors. As previously stated, the Tobit model has the advantage of eliminating bias and inconsistency when estimating unknown parameters, making it a superior option for assessing the main drivers of the GTFP (Kuang et al., 2020). Consequently, the Tobit model is becoming more popular for resolving methodological difficulties in the second stage using the DEA approach (Debbarma et al., 2021). Thus, we determined that Tobit regression is a superior (or more specific) methodology in this study, with more significant theoretical and practical implications.
Results of the estimation results
Dependent variable=Average value of MLPI (Table 3) | ||||
---|---|---|---|---|
Explanatory variables | (a) OLS Model | (b) Tobit Model | ||
Coefficient | (t-statistics) | Coefficient | (t-statistics) | |
Exporting firm | ||||
Product | .4541498*** | (3.76) | .6140304*** | (4.30) |
Patents | .3526499*** | (2.86) | .3644527*** | (2.79) |
R & D | -.0000339*** | (-5.15) | -.0000484** | (-2.42) |
Process | .086342 | (1.06) | .1425933 | (1.58) |
HCQ | .2517317* | (2.02) | .4114171** | (2.53) |
EC | 2.257944 | (1.14) | 3.417538 | (1.60) |
_cons | -2.35032 | (-1.22) | -3.869644* | (-1.81) |
Non-exporting firm | ||||
Product | .0027143 | (0.02) | .0179122 | (0.11) |
Patents | .1061732 | (0.57) | .1428297 | (0.74) |
R & D | .3509172** | (2.36) | .4467681** | (2.75) |
Process | -.0000108 | (-0.41) | -.0000132 | (-0.48) |
HCQ | .4928611*** | (3.07) | .5726294*** | (3.39) |
EC | 3.34505 | (0.57) | 4.506676 | (0.74) |
_cons | -3.422366 | (-0.59) | -4.616426 | (-0.75) |
Note: ***, **, and * indicate the 1%, 5% and 10% levels, respectively.
The main drivers of TC on GTFP growth are presented in column (b), which shows that the variables affecting the GTFP are different for exporting and non-exporting firms. The results for exporting firms show that product innovation contributes to fostering technological innovation and promoting GTFP, with the highest coefficient and significance level of 1%. This suggests that a firm's ability to enter a foreign market and compete successfully against a local offer (which inevitably has on-site advantages) is closely related to a broader product range and the availability of a novel product (Nassimbeni, 2001). For non-exporting firms, HCQ is regarded as an important TC factor that promotes the GTFP. This implies that areas with a high concentration of human capital are more likely to attract inflows of advanced technologies. When human capital is promoted, it is easier to promote the emergence of technological progress, which results in an improvement in the quality of manufacturing supply (Lei et al., 2013). Meanwhile, the impact of R&D expenditure shows conflicting relationships between exporting and non-exporting firms, such that the R&D coefficient is negative for exporting firms and significantly positive for non-exporting firms. This pattern implies that exporting firms tend to derive no productivity gains from R&D investments compared with non-exporting firms during the study period. This may be because most exporting firms are under pressure from the global market to reduce CO2 emissions; therefore, they often invest extensively in R&D activities to mitigate CO2 emissions and improve productivity. However, because of their extensive investment in R&D technology, Indian local market conditions (legacy systems) may not fit in unison, resulting in relatively low performance despite the high level of facilities. Consequently, with more investment in high levels of R&D technology, they may encounter issues with the link to the legacy system. Thus, this type of heavy investment in R&D may have a detrimental impact on the reduction in CO2 emissions for exporting firms. Therefore, R&D should be conducted in a much more field- or performance-oriented manner.
Conclusions and policy implicationsThis study proposed that exports could be the best solution for businesses to tackle climate change by adopting the efficient and effective access of information technology through “learning by exporting” effects. This is because as entry into export markets improves firms’ agility to adopt new norms such as climate change, technological innovation, and exposure to much more severe global competition. This study then used the DDF and MLPI to estimate the GTFP of 52 manufacturing firms (28 exporting and 24 non-exporting firms) in India between 2012 and 2020.
The main findings are summarized as follows. First, based on MLPI, the GTFP performance of exporting firms increased by approximately 7.9% on average, indicating that a “learning by exporting” effect exists in India and that exporting firms could help tackle climate change in India. Non-exporting firms experienced a 0.7% decrease in GTFP performance, implying that non-exporting firms should learn from exporting firms by exploring overseas markets and participating in exports, which will automatically improve their efficiency. Second, by decomposing the MLPI, we identify the main driving factors that promote productivity growth. The MLPI decomposition reveals that TC is larger than EC in both groups, showing that technological innovation is the main driver of GTFP growth. This finding suggests that the adoption of new technologies is critical for industrial productivity. More importantly, Indian policymakers should pay more attention to the concepts and management approaches used by frontier firms (exporting) to promote current energy technology innovation and urge inefficient firms to learn from them to strengthen their ability to innovate independently. Third, to some extent, success or failure in improving the GTFP largely depends on whether we can achieve breakthroughs in technological innovation (Poonia et al., 2021). The Tobit regression analysis in Table 6 (column (b)) identified several factors with strong explanatory power for TC in Indian manufacturing firms. The results show that exporting firms with new product development are most likely to promote GTFP growth, with a significance level of 1%. Based on these findings, policymakers should encourage exporting firms to invest more in environmentally friendly products related to innovation by offering financial incentives, such as tax incentives, for developing green products to enhance industrial productivity. Among non-exporting firms, TC is best characterized by HCQ. This suggests that non-exporting firms should continue to promote HCQ by hiring more skilled labor and encouraging them to expand their knowledge-based skills to introduce more advanced production and management technologies to boost the innovative effect of promoting GTFP.
Based on the study findings, we propose that future emission reduction policies in India be more field- or performance-oriented while also supporting the adoption of innovative technology for environmental preservation and energy conservation. A strong promotion policy, such as a tax incentive, could be more effective for firms to export because it helps improve nationwide productivity. There is no panacea to cure all the problems. However, “prudently” harmonized combinations of regulation and promotion should be adopted to accomplish sustainable development.