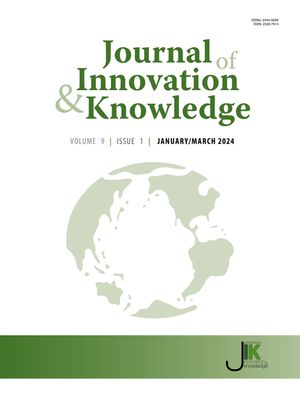
Edited by: Abbas Mardari
More infoAs a new strategy to combat global climate change and achieve sustainable development, a marine low-carbon economy has gradually gained attention in recent years. A variety of reasons are fueling the creation of a marine low-carbon economy. The digital economy (DE) has demonstrated a significant new thrust in the low-carbon production and consumption sectors. This study measures the effect of the DE on the marine low carbon (MLC) level using the mediation effect model and spatial Durbin model in 11 marine provinces of China from 2007 to 2018. The empirical results show that the impact of regional heterogeneity of the DE on MLC presents a U-shaped relationship. The mediation effect shows that the DE can affect MLC through industrial structure updates and innovation efficiency. After considering the geographic, economic, and spatial distance matrix, the DE has a positive spatial spillover effect on MLC in the adjacent areas. Therefore, this study has a depth implication for promoting MLC by the DE with its spatial spillover driving through the marine industry updates and innovation efficiency.
Many blue carbon sinks in the marine environment may efficiently absorb carbon dioxide in the atmosphere and ease environmental pollution (Fre et al., 1992; Masakazu et al., 2018; Michael et al., 2022). As estimated by the United Nations, more than half of the world's natural carbon sinks are provided by marine species, such as phytoplankton, bacteria, salt marsh plants, and mangroves (Clemons et al.,1993; Abhijit et al., 2015; Tang et al., 2018). Half of the annual emissions from the global transportation sector are captured and stored by blue carbon sinks and estuaries, storing 8.7–16.5 million tons of CO2. If the destruction and degradation of marine ecosystems can be prevented further, 3%–7% of CO2 emissions from fossil fuels (or approximately 270 million tons) will be offset for the next 20 years. Hence, all nations’ key priorities include developing a marine low-carbon economy, safeguarding the marine ecology, and realizing marine circular growth (Li et al., 2021; Zhou et al., 2021).
In 2003, the British government proposed a “low-carbon economy” in “Our Energy Future: Creating a Low Carbon Economy” (Foxon et al., 2007). The core of low-carbon economic development is a model of economic growth based on low energy consumption, low pollution, and low emissions, as primarily manifested in the improvement of energy efficiency, the optimization of energy structure, and the rationalization of consuming behavior (Wang et al.,2022; Wang, Wang & Li, 2022). At present, the marine economy is also under pressure to reduce carbon emissions as marine-related businesses, such as marine chemical, salt, fishing, shipbuilding, transportation, oil and gas, coastal sand mining, and marine engineering construction, use much energy and negatively impact the environment (Aradhana, 2008; Bagoulla et al., 2020).
China, the largest developing country and the second-largest economy in the world, has an extensive coastline and a wealth of marine resources. As a result, its marine economy is one of the most productive in the world. Compared with other developing countries, China's research on marine low carbon (MLC) has aroused great attention with the implementation of marine power since 2003 (Su et al., 2021). In 2003, China created the Outline of the National Marine Economic Growth Planning, publishing the sector's growth strategy and spatial planning. After that, China's marine economy achieved world-renowned achievements. According to the “China Marine Economic Statistical Bulletin,” China's marine gross domestic product (GDP) will be close to $140.1 billion in 2021, up 8.3% from the previous year and 15.0% of the country's overall coastal GDP.
Nevertheless, scholars proposed that MLC pays a considerable cost to resources and the environment. The main effects include severe depletion of fishing resources, deterioration of marine biological ecosystems, and inefficiencies in the development of marine resources, severely impeding China's marine economic growth (Okoyen et al., 2020; Yang et al., 2020). Therefore, a MLC should be developed to maintain the growth of marine ecosystems (Narissa Bax et al., 2021). Accordingly, our work builds on the exploration of the promotion of MLC.
Concerning the factor affecting the MLC, the digital economy (DE) is becoming a new driving force for economic and social development with the continuous development and broad application of digital technologies, such as the Internet, big data, cloud computing, and blockchain. As the fifth largest factor of production alongside traditional aspects of land, labor, capital, and technology, digital technology can make up for the lack of marine industry development to a certain extent. The DE has penetrated all aspects of marine production activities with new changes to the development of the marine industry, such as marine fishery, marine high-end equipment manufacturing, marine pharmaceutical industry, and other related marine industries. The development mechanism of MLC by the DE remains relatively unclear. Most of them are limited to a particular area or a specific aspect, such as marine fishery and marine transportation (Agrifoglio et al., 2017; Hilde et al., 2018; Hilde et al., 2020). Moreover, the causal effect of the DE on MLC was not examined precisely either. Based on this, this article built a comprehensive level index of the DE using marine provincial panel data from 2007 to 2018, adopted the marine green total factor productivity, and methodically analyzed the effect of DE on MLC.
The contributions of this research are as follows. First, the DEA-Malmquist model was utilized to calculate the total factor productivity of the low-carbon maritime economy. In contrast to traditional marine comprehensive factor productivity accounting, this model considered both the input of energy and the decrease in carbon dioxide emissions, successfully compensating for the inadequacies of conventional marine economic efficiency accounting. Second, the DE level was evaluated from these factors consisting of its infrastructure, application, industry development, and development environment to overcome the limitations of conventional unilateral measurement. Third, few studies integrated the DE, marine industrial structure, innovation capabilities, and MLC in a framework. This study innovatively incorporates them to deepen existing literature and research. Finally, most research identifying the factors influencing MLC lacked the consideration for spatial spillover effect, particularly from the DE.
The structural content of this paper is as follows. Section 2 reviews the theoretical logic of the DE and MLC with some theoretical assumptions. Section 3 presents the methodology, including the construction of the model, the description of the variables, and the data source. Section 4 is the empirical analysis of this study, including heterogeneity analysis, mechanism of action, spatial effect, and robustness test. Section 5 presents the results, and Section 6 discusses the effects of DE on MLC. Finally, Section 7 concludes the study.
Literature reviewThe topics of DE and MLC have attracted the focus of academics. However, the connection between the two needs further investigation, and research on whether the DE can significantly contribute to China's MLC expansion is still ambiguous. Therefore, investigating this relationship further is reasonably practical.
First, various calculating approaches for MLC research are proposed to analyze this development efficiency. Alexa et al. (2021) investigated the influence of environmental pollution on economic development, emphasizing that ecological protection should be paid attention to in developing marine economic growth. Environmental protection was included as a primary indicator for the first time when Semenov (2008) extended the maritime economic development evaluation index system. Kong and Liu (2021) proposed that institutional investors in coastal cities focus on carbon financing initiatives to reduce carbon dioxide emissions and enhance marine city ecological development. In addition, more studies are being done on carbon emission effectiveness. Litavcov and Chovancov (2021) proposed using CO2 intensity as an indicator to evaluate a country's or region's carbon emission efficiency, namely, the quantity of CO2 emission per unit of GDP. Ramanathan (2006) analyzed the relationship between energy utilization, carbon emissions, and GDP using a data envelopment analysis (DEA) approach, showing the historical pattern of greenhouse gas emissions efficiency. Zhou et al. (2010) compared the effectiveness of the environmental DEA method's carbon dioxide emission reduction in 18 major nations. Zhao and Zeng (2020) calculated the green economic efficiency of 30 provinces from 2008 to 2017 in China by using the super efficiency slacks-based measure approach. The influencing elements of green economic efficiency were experimentally evaluated using the spatial Durbin approach. The low-carbon economy research currently focuses on the theory and methods of the low-carbon economy, including the variables that impact it and on evaluating its efficiency. Most DE studies concentrated on the intellectual underpinnings of digital innovation and its outcomes (Hai et al., 2022).
Second, research has been done on the effect of sustainable development with the DE as it continues to expand. Most academics agree that digitization may improve long-term economic growth. Osamu (2005) emphasized that global digital networks may be crucial in the long run if the state of the world's environment worsens, linking the world and fostering new social norms that transcend nationalist concerns. Martynenko and Vershinina (2018) believed that resource conservation and the long-term development of nations, regions, and the entire human race might be possible as production becomes more ecologically modernized. According to Li et al. (2022), the DE may complement green development. The DE can successfully support the global economy's green transition. In addition, digital technology may enable the green economy to achieve sustainable, low-carbon, and long-term development (Ernest et al., 2021).
However, other academics asserted that although the Internet is good for the environment, it also harms the ecological system. Shvakov and Petrova (2009) discovered that the DE has not aided but hampered the growth of a green energy-saving economy through data analysis of the top 10 nations in the world's economic development in 2009. In addition, the DE's expansion rates should be curbed to achieve sustainable economic growth. Gozal et al. (2022) corroborated the moderating influence of a country's institutional structure on the connection with digitalization and sustainability practices by analyzing 5015 enterprises from 47 countries across 10 industries from 2014 to 2019. Li et al. (2021) used the Solow growth model to discover an inverted U-shaped correlation between CO2 emission and DE for 190 nations from 2005 to 2016.
According to the research mentioned above, most studies on MLC are qualitative at this point. Instead of using theoretical or quantitative models, they typically conduct a descriptive study to examine how the DE impacts the environment. Thus, how can the low-carbon efficiency of the digital and marine economies be assessed more scientifically and effectively? What is the effect of the growing DE on marine CO2 emissions? Is there a nonlinear relationship between DE and marine CO2 emission? Does this relationship still hold under consideration of spatial effects? People are becoming increasingly interested in how to transform and upgrade industries with high levels of energy consumption and emissions. Is it conceivable that enhancing the marine industry structure and promoting technological innovation inside the DE could boost the effectiveness of the marine low-carbon economy? It has become an urgent issue that must be addressed. Based on this, using panel data covering 11 coastal provinces from 2007 to 2018, this research explored the influencing channel of DE in TMLC utilizing marine green total factor productivity.
Methodology and materialsEmpirical modelTo test the above theoretical hypothesis, we first construct a direct impact mechanism model:
where DEA−Malmquisi,t represents MLC in region i at period t that digi,t denotes the DE development level and Controlsi,t encompasses a set of control variables with the individual fixed effects μi and the random disturbance term εi,t.According to H2, H3, and H4, this study constructs a mediation effect model:
In Eq. (2), the DE has a significant impact on the marine industry advancement and innovation efficiency (IE) of the intermediary variables after incorporating the marine industry structure and IE into Eq. (3). If the regression coefficient is sufficiently decreased, then the design of the maritime industry and the effectiveness of innovation will play a whole or partial mediating role. These can be generally divided into two types, which are as follows: When the regression coefficient decreases significantly but not zero, the marine industrial structure and IE are partial mediating effects. Then, when the regression coefficient drops to zero, the marine industrial structure and IE are completely mediating effects.
Existing studies showed that the barriers between regions gradually shrink with time, and the flow of factors and products between neighboring provinces and cities is more accessible, gradually reducing the scale of spatial spillover effects between regions. Environmental pollution affects the local ecological environment and adjacent areas, and space has an evident correlation.
Based on this, this research develops a spatial measurement model identifying the effect of carbon emissions by DE.
where Wij is the spatial panel weight matrix, where the 0–1 matrix was used, ρ and δ are the spatial autoregression and autocorrelation coefficient; respectively, Xit is the explanatory variables of province i in year t, including the core explanatory variables, such as the primary term of the DE, the quadratic term of the DE, and other relevant control variables. In Eq. (4), εit is the spatial error autocorrelation term and λi,t is the random interference term. The typical spatial econometric models are spatial autoregressive (SAR), error (SEM), and the Durbin model (SDM). When ρ in Eq. (5) is not zero and θ and δ are both zero, the model is SAR; when ρ and θ are zero and δ is not zero, the model is SEM; when ρ and θ are not zero and δ is zero, the model is SDM.Measurement and descriptionExplained variablesThis study applies the DEA-Malmquist productivity index method to calculate the MLC. When determining total factor productivity, a DEA model and the Malmquist index are applied as measuring equipment. The principle of linear programming is first used to obtain the distance function and then constructs the Malmquist exponent through the ratio of the distance functions in different periods. Färe et al. (1997) created the DEA-Malmquist to avoid reference to its random selection to calculate the productivity index across regions under constant returns to scale (CRS). This method divides total factor productivity (TPF) into technical efficiency index (Effch) and technological progress index (Tech). Moreover, the technical efficiency index (Effch) is further divided into pure technical efficiency index (Pech) and scale efficiency index (Sech). The model is constructed as follows:
In Eq. (6), x and y represent input-output indicators, respectively (Table 1), Dt and Dt+1 define the distance function between periods t and t+1; when Mt > 0, the change in total factor productivity (TPF) is a positive from periods t and t+1, indicating that total factor productivity (TPF) increases. Conversely, when Mt<0, the total factor productivity (TPF) declines.
The essence of the MLC is an economic development approach that applies the low-carbon concept to the marine industry's development process. Low energy consumption, low emission, and low pollution features in the marine industry are critical in enhancing the utilization efficiency of marine resources and optimizing the energy structure. Its central themes include technical innovation, institutional innovation, the transformation of development paradigms, and the accomplishment of the circular development of the economy and ecological development. Therefore, the input indicators of this study select marine fixed assets input, labor input, and energy input. Compared with the output index, which only examined the gross marine product GDP in the past, this study considers the undesired output and examines the emission of pollutants. The emission of pollutants mainly includes gas, solid, and liquid emissions. Table 1 shows the specific indicators.
According to the index system in Table 1, the entropy weight method was used to calculate the MLC of 11 coastal provinces in China from 2007 to 2018. Fig. 1 shows the calculation results.
Explanatory variablesIn this study, the evaluation index of DE can be constructed from four aspects consisting of infrastructure, application, industry development, and development environment. The entropy weight approach was used to assess the digital economic growth of 11 Chinese coastal regions from 2007 to 2018 (Nanxu et al., 2022; Le, 2022).
According to the index system in Table 2, the entropy weight method was used to calculate the DE of 11 coastal provinces in China from 2007 to 2018. Fig. 2 shows the calculation results.
Evaluation index of the digital economy.
The DE is the main economic form after the agricultural and industrial economies. It views data resources as the fundamental component; contemporary information networks as the primary carrier; the use of information and communication technology; and the digital transformation of all members as essential driving forces to promote a just and new economic form with a more unified efficiency (Bukht et al., 2018). The DE can effectively reduce resource input and environmental pollution and improve ecological efficiency in marine economic development by stimulating IE, optimizing industrial institutions, and improving production efficiency. Few scholars previously integrated the DE, marine industrial structure upgrading, technological innovation, and the efficiency of MLC in the framework. The DE may have an indirect effect through industrial structure upgrades and technological innovation. Additionally, the spatial spillover effects of the DE are apparent positively in MLC. This research investigates the influence mechanism and effect of DE on MLC and thus makes the following assumptions:
Direct effect of the digital economy on marine low carbonThe rapid growth of digital technology, new industries, work frameworks, and models continue to emerge, boosting the rapid development of the DE. The result of computer technology has been accelerating since the 1990s. Many new economic systems, including the DE, have emerged because of developments in fields such as artificial intelligence (AI), blockchain technology, and 5 G Information technology. Neo-Schumpeterian economic growth theory holds that endogenous research and development (R&D) and innovation are the core driving forces for technological progress and economic growth, and the essence of economic growth is innovation. The so-called “innovation” means “establishing a new production function,” introducing a “new combination” of production factors and production conditions that have never been seen before into the production system, thereby achieving a qualitative change in economic growth (Hanusch et al., 2014; Agnieszka et al., 2022). The DE benefits the environment by identifying, selecting, filtering, storing, and exploiting big data to improve resource efficiency. At the moment, scholars mainly focused on the DE's effect on reducing emissions at three levels of analysis: macro, meso, and micro.
First, from the macro-level perspective, the government can quickly and precisely grasp the dynamic information of the energy market using digital technology and optimize resource allocation through macro-control measures. On the one hand, the government can control the energy supply by controlling prices and providing subsidies. On the other hand, the government can also better control unlimited energy use through the digital operation of the carbon emissions trading market, effectively controlling total carbon emissions. Second, from the perspective of the mesa level, digital technology facilitates industrial structure optimization and upgrade at the mesa-scopa level. Digital technology speeds up the transition of production elements from low-efficiency to high-efficiency sectors, improves energy efficiency, reduces carbon emissions, and promotes the industrial structure. Finally, from the micro-level perspective, the application of digital technology can optimize the governance technology of enterprise carbon emissions and achieve real-time collection, detection, transmission, and analysis of energy data by accurately grasping energy flow data and guiding energy factors allocated efficiently. With the support of digital finance, corporate financing constraints can be reduced. Resource mismatches can be alleviated by improving energy utilization efficiency, promoting total green factor productivity, and reducing carbon emissions. In addition, from the energy conservation perspective, the DE breaks regional boundaries, breaks through time constraints, accelerates the flow and production of factors, saves energy consumption caused by space and time factors in production and life, reduces energy consumption rates, promotes the improvement of energy utilization efficiency, and then reduces carbon emissions.
However, many academics observed that the influence of DE on the environment has positive “green blind spots,” which have negative external impacts on the environment and lead to increased carbon emissions. This influence is most apparent in two different ways. First, with the widespread use of digital technology for resource extraction, the scale of resource extraction has increased, resulting in excessive energy consumption. Meanwhile, the digital industry needs to consume electricity, such as telecommunications, software, emerging technology services, and the Internet. In China, the proportion of coal-fired electricity is currently relatively high, and the consumption of electricity means an increase in coal consumption, increasing carbon emissions. According to Li et al. (2021), the DE advancement will assist firms in rebuilding production equipment during the phase of economic development, allowing businesses to increase production by increasing resource extraction and energy consumption, increasing carbon emissions. When a strong economy advances to a higher level, the show is constant, and digital technology and scale reduce the cost of pollution management, resulting in lower carbon dioxide emissions, indicating the DE's nonlinear influence on carbon emissions. To this end, this study proposes H1:
Hypothesis 1: The effect of the digital economy on marine low carbon efficiency is a positively U-shaped nonlinear correlation.
The DE indirectly impacts low-carbon efficiency through the industrial structure transformation (IST). The development of platform economy, sharing economy, and other formats in the DE is conducive to strengthening market competition, accelerating the logical flow and optimal combination of resource elements, and improving the coupling between industrial structure and resource element ratio. Meanwhile, the fast growth of Internet trading platforms has overcome traditional transactions’ time and location constraints, accelerating the cross-regional movement of resource elements. The area of resource allocation will also be broadened, and resources will flow into sectors with better production efficiency, improving the industrial structure. As a result, industrial structure modification may significantly cut pollutant emissions (Mrtin & Manfred, 1997). The optimization of IST is reflected in the improvement of resource utilization efficiency, which is beneficial to reducing the waste of resources and significantly improving environmental quality. Meanwhile, the optimization of IST means that the proportion of technology-intensive industries increases. These intense industries are clean, with high efficiency and low energy consumption, significantly reducing environmental pollution emissions.
The DE seems to impact low-carbon development through IE indirectly. According to Miller and Wilsdon (2001), the DE symbolizes a technological revolution and is a primary determinant of technological innovation. The digital infrastructure has been continually enhanced as the DE has grown. The widespread use of digital technologies, such as search engines and cloud storage, can help enterprises, universities, and other innovative institutions get and manage information while reducing external information search and management expenditures. In addition, the DE breaks the barriers to information flow. This case enables a more timely exchange of knowledge among innovative entities and optimizes the management efficiency of R&D teams. It also enables innovative entities to capture market feedback on products and services fully, understand the market's response to new product R&D needs, and promote the improvement of IE.
Hypothesis 2: The digital economy promotes industrial structure transformation and innovation efficiency.
Hypothesis 3: Industrial structure transformation and innovation efficiency promote marine low carbon efficiency.
Hypothesis 4: The digital economy can achieve marine low carbon efficiency by industrial structure transformation and innovation efficiency.
The DE is characterized by two essential qualities: a reduction in the geographical barriers between locations and an enhancement of the links among different regions’ economic activities (Ding et al., 2022). A substantial geographical link in marine economic activity also exists, and regional barriers are gradually being reduced.
The expansion of the DE has made the transfer of marine resources and merchandise across nearby locations more straightforward, and the impact of spatial spillover effects in these regions is evident. The rise of the DE has made transferring marine resources and merchandise across nearby locations more straightforward, and the impact of spatial spillover effects in these regions is evident. The DE's growth has accelerated the circulation of marine resources and enhanced aquatic innovation activities, which has a geographical spillover effect on regional economic development. Therefore, carbon emissions affect not only the ecological mitigation of the local area but also the adjacent areas, and an apparent spatial correlation exists.
Hypothesis 5: The digital economy has the potential to make a contribution to the marine low carbon in surrounding regions through spatial spillover effects. Fig. 3 shows the proposed framework.
- (1)
Industrial structure transformation
This research uses the grading coefficient of IST to represent changes in the industrial structure and the level of evolution of the industrial structure (Xiao & Peng, 2021; Romano & Traù, 2017).
In Eq. (7), ISTit represents the index of the IST in province i in year t; Ritm represents the proportion of the m industry's GDP in province i in year t; R represents the three industries; The value range of IST is [1, 3]; the closer the IST calculation result is to 3, the higher the degree of IST.
- (1)
Innovation efficiency
China's evaluation approach for regional innovation capability focuses primarily on five areas of regional innovation capability. These components include knowledge generation and acquisition, innovation in the enterprise, innovation in the environment, innovation performance, and innovation environment. Among them, IE estimates a region's capacity to enhance innovation concerning economic growth (Lyu et al., 2022). This study mainly examines the role of IE in developing a MLC.
Control variablesIn collaboration with the previous research literature, this article introduces the consumption level of urban residents, labor level, marine fixed asset investment, and marine local financial expenditure as control variables. The specific meanings are as follows:
- (1)
Economic development level. Environment Kuznets believes that in the early stage of economic development, economic growth will lead to the deterioration of the environment. Moreover, with the continuous development of the environment, the domain will be improved. This study uses per capita GDP to represent the level of economic development (Hui et al., 2021).
- (2)
Consumption level of urban residents. Consumption of urban residents refers to the per capita number of residents used for living expenses, which reflects the quality of people's material life; residents’ living consumption leads to an increase in energy consumption, which may further increase carbon emissions (Glen, Christopher, Guan & Hubacek, 2007).
- (3)
Financial development level. The Douglas production function believes that capital is necessary for social production and operation activities. In the process of production and expansion of reproduction, increasing capital investment can increase investment in technology R&D, thereby achieving the purpose of improving resource efficiency. This process can also reduce carbon emissions and form the “emission reduction effect” of financial products (Wang et al., 2020).
- (4)
Government intervention GOV. Government intervention plays a vital role in solving energy and environmental problems in China. Implementing environmental policies and investment in pollution control is inseparable from government intervention. However, excessive government intervention is not conducive to improving carbon emission efficiency. This study uses the ratio of the government's financial expenditure on the ocean to the ocean's GDP to represent the degree of government intervention (Lin et al., 2021).
- (5)
Development level of urbanization. The urbanization rate mainly refers to the proportion of the resident population in urban areas to the resident population in the region. Urbanization can realize the efficient allocation of factors in the production space by adjusting the scale, structure, and layout of different functional areas, thereby improving the efficiency of industrial development and enterprise production (Qiang et al., 2022).
This study utilizes panel data from 2007 to 2018 covering 11 coastal provinces information for empirical research. Table 3 shows the descriptive statistics of variables from the empirical study “Innovation Capability Evaluation Report,” “China Regional Innovation Capability Evaluation Report,” and other relevant statistical yearbooks.
Descriptive statistics of core variables.
According to the data, the average level of MLC is 1.015, with a maximum of 1.888 and a minimum of 0.626. This result means that the rate of MLC varies substantially among locations, confirming prior study findings.
ResultsEffect of the digital economy on marine low carbonFirst, the primary term of DE is included in the regression analysis. Table 4 presents the specific regression conclusions after controlling for other variables, such as time and individuals, that may impact the degree of MLC. Among them, model 1 is the regression of the DE's primary term, and model 2 is the DE's immediate term considering control variables. According to the regression results, the regression coefficients of the DE are all positive, but none of them have passed the significance test. This finding demonstrates that DE positively affects the efficiency level of MLC, but this linear relationship has not been verified in statistical models. Models 3 and 4 add the DE plus quadratic term to the model to examine whether the DE affects MLC in a nonlinear relationship. The DE passed the significance test, and the result of the regression coefficients was positive, demonstrating a significant U-shaped correlation between DE and MLC (Fig. 4). Fig. 4 shows that when the DE is 24.95, an inflection point exists. That is, when the DE is less than 24.96, it will hinder the development of MLC; when the DE is more significant than 24.96, it will promote the development of MLC.
Impact of DE on MLC.
Note: (1)*p < 0.1, **p < 0.05, ***p < 0.01; (2) The T-statistic was shown in parentheses.
The main reason may have been that technical advances enabled by the DE would allow firms to rebuild production equipment at an early stage of economic development, increasing the output by increasing resource extraction and energy consumption and thereby increasing unit carbon emissions. However, when the growth rate of DE is high, the production of enterprises is steady, which reduces the number of pollutants discharged into the environment.
Among the control variables, the coefficients of per capita GDP and per capita consumption are negative, which means that increasing per capita GDP and per capita consumption will diminish the MLC. As the coefficients for GDP per capita and consumption per capita are negative among the control variables, an increase in GDP per capita and consumption per capita will lead to a decrease in the low-carbon efficiency of the marine industry. Therefore, when the per capita income level increases, the consumption of marine aquatic products for individuals and families will increase. The increase in household wealth will further increase the demand for marine activities, such as recreational fishing and marine transportation. However, this result did not pass the significance test, demonstrating that the signal is not apparent. Financial development has a substantial beneficial effect on carbon emission efficiency primarily because digital finance can effectively ease the problem of information asymmetry and achieve the optimal deployment of financial resources, enhancing emission efficiency. The local fiscal expenditure is significantly positive, indicating that government support can improve the low-carbon efficiency of marine. The possible reason is that government support shows a clear tendency to reduce emissions. The increase in urbanization has a negative effect mainly because urban inhabitants consume more energy per capita than rural residents. Therefore, the increase in the urbanization rate reduces carbon emission efficiency to match reality.
Further expansion: regional heterogeneityConsidering the unbalanced development of DE in various regions in China, this study is divided into three major marine economic circles for consideration: Model (1) represents the northern marine economic circle, which consists primarily of Liaoning, Shandong, Tianjin, and Hebei; Model (2) represents the central marine economic circle, which consists primarily of Jiangsu, Shanghai, and Zhejiang; and model (3) represents the southern marine economic circle, which consists primarily of Guangdong, Guangxi, and Hainan. Table 5 shows the result.
Regional differences.
Note: (1)*p < 0.1, **p < 0.05, ***p < 0.01; (2) The T-statistic was shown in parentheses.
Table 5 shows that the DE significantly influences the growth of the marine economy in all three marine economic circles. However, in terms of the degree of impact, the southern marine economic circle has the highest influence coefficient, followed by the center section, and the smallest in the northern part. This phenomenon may be because the DE in the southern marine economic circle develops on a larger scale, which may better cut the transaction cost of marine economy operation, increase marine economy productivity, and promote marine economy reform. The DE and MLC are in a virtuous circle.
The impact of the DE on MLC in the southern marine economic circle is more significant than that in the central marine economic process. The influence of the DE in the south of the marine economic circle is far more important when compared with its effect on low-carbon efficiency in the central marine economic process. The primary reason might be that the magnitude of maritime economic growth is insufficient. The northern marine economic circle's DE development level cannot match the economic size. The vast economic development strategy is not favorable for developing MLC.
Mediation effectFollowing that, this article adopts the previously indicated mediation effect to examine if IST and IE have a mediation impact between the DE and MLC. Table 6 presents the displays. Model (1) explores the effects of the DE on IST, which is meaningful at the 10% significance level after controlling for time and individual factors. The DE and its square results are highly significant, suggesting that the DE has an excellent U-shaped link with IST. Model (2) includes IST and the DE to analyze the influence on MLC. The DE's impact on MLC remains a substantial U-shaped correlation, and IST has a significance level of 5%. The model coefficients of the DE on low-carbon efficiency all decreased after including the intermediate variable IST, showing that the IST played a partly intermediary function. That is, the DE would indirectly impact MLC by altering the IST. As shown in Fig. 4, the mediation effect of the IST shows that beyond this inflection point, the higher the degree of DE, the higher the IST, and the higher the degree of MLC.
Mediation effect.
Note: (1)*p < 0.1, **p < 0.05, ***p < 0.01; (2) The T-statistic was shown in parentheses.
Model (3) examines the influence of the DE on IE, showing that after controlling for time and personal effects, the impact of the DE on IE is substantial at the 1% significance level. The square of the DE and the DE is highly positive among them, showing that the DE has a positive U-shaped correlation with IE. Model (4) includes the DE and IE in the model to assess the influence on IE. The DE's impact on MLC remains a substantial U-shaped correlation, and IE is significant at the 5% significance level. The model coefficients of the DE on MLC all decreased after including the intermediate variable IE, showing that the IE played a partly intermediary function; that is, the DE would indirectly impact MLC by altering the IE. As seen from the mediation effect diagram of IE in Fig. 5, beyond this inflection point, the higher the degree of DE, the higher the IE, and the higher the degree of MLC.
Spatial spillover effectsSpatial correlation testFirst, Moran's scatter plot is used to analyze the spatial correlation between cities, and then, the rationality of the spatial analysis is judged. As the growth of MLC is asymptotic, the spatial characteristics of MLC should be measured only in the last year (i.e., only the spatial characteristics of MLC in 2018 and the spatial correlations of MLC can be identified). In spatial characteristics, the length of distance and the level of economic development are closely related to MLC. The length of distance affects the accessibility of transportation and talent flow, whereas the level of economic development affects the flow of capital, technology, and talent to a certain extent.
To this end, this study uses geographic and spatial distance as a matrix to report the Moran to scatter plot of MLC in 2018. Fig. 7 shows the results. The abscissa is the MLC after the standardization of each region, and the ordinate is the MLC after the standardization of adjacent areas (A is geographic distance; B is economic distance). In the partial Moran scatter plot, if a city is in the first quadrant, then its economic development level is high, and it is surrounded by high-value cities (regions); if it is in the second quadrant, then its economic development level is low but is surrounded by high-value areas. If it is in the third quadrant, then its economic development level is low and is surrounded by low-value cities (regions); if it is in the fourth quadrant, then its economic development level is high but is surrounded by low-value parts.
Fig. 7 shows that the Moran indices of geographic distance and economic distance are 0.1008 and 0.2085, respectively, and the null hypothesis is rejected at the 5% level. This result shows that MLC exhibits an evident spatial correlation. Among them, Hebei (HB), Shandong (SD), Jiangsu (JS), Liaoning (LN), Fujian (FJ), and Hainan (HN) are in high and high aggregation areas. This result indicates that in addition to their high MLC, the MLC in adjacent regions is relatively high. On the other hand, Guangxi (GX), Hainan (HN), and Guangdong (GD) are in low and low agglomeration areas, indicating that although these areas have a low level of MLC, their adjacent regions have low levels.
According to the distribution of the center of gravity of MLC and DE in 2018 (Fig. 8), as the eastern marine economic circle is located in the Yangtze River Delta region of China, China's Yangtze River Delta region has a high degree of integration. To a certain extent, this case promotes the accumulation of marine green resource elements, showing a “center-periphery” feature. Compared with the southern and northern marine economic circles, the development of DE and MLC in the eastern marine economic zone shows a higher level. Looking at the spatial layout of marine economic growth, China's MLC is facing a problem. Thus, a significant spatial correlation exists in China's MLC, and the spatial measurement Moran is suitable to use for the next step.
Spatial measurement modelIn addition, LM, Robust, Wald, and LR tests were utilized to determine the spatial empirical model. The test result showed the null hypothesis that the SDM model degenerates into the SAR model (Table 7). Therefore, the setting of the SDM model is reasonable. Furthermore, the Hausman statistic notes that negative values do not reject the random effect null hypothesis. Thus, this study chooses the random effect SDM model.
Empirical analysis of spatial effectsAccording to Moran mentioned above in the scatter diagram of MLC in 2018, a spatial correlation can be observed in the growth of MLC. Moreover, the above test results of the mediation effect show that the DE promotes the IST, stimulates the IE of coastal areas, and then produces the MLC. As China's coastal regions are interconnected, the MLC in each location can guide the transformation of marine economic activities in the surrounding areas, changing the entire space system. Therefore, this chapter uses the geographic and economic distance as the weight matrix and adopts the spatial Durbin model to test the spatial spillover effect of the DE on the MLC. Table 8 shows the results.
Spatial Doberman model estimation.
Note: Robust standard errors are in brackets; ***, **, and * indicate significance at the 1%, 5%, and 10% levels, respectively.
From the results in Table 8, regardless of whether geographic distance or economic distance is considered in the weight matrix, the indirect influence coefficient of IST and IE on MLC is insignificant, which shows that MLC is not affected by adjacent IST, the driving role of IE. In other words, MLC is only driven by IE elements in specific coastal areas (e.g., its area), but the activity space of marine industry and commerce will not have a diffusion effect on MLC. When controlling IST and IE simultaneously, only the coefficient of IE on the direct impact of MLC is significant at the 10% level. Compared with the IST, the direct effect of IE on marine MLC is more prominent, which may be because of the “self-mechanism” effect of IE itself. The accumulation of IE can promote the MLC due to a differentiated accumulation.
In terms of the impact of the DE, when the square term of the DE is considered, the DE and DE2 are not significant in terms of direct and indirect effects, but they are consistent in the sign of the indirect effect coefficients. This result shows that in the spatial activity system of coastal areas, no spatial U-shaped relationship exists between the DE and MLC. However, general spatial equilibrium is manifested in the positive diffusion effect of the DE on the MLC in adjacent provinces. Therefore, the MLC is gradually rising due to the spillover of the neighboring DE.
This conclusion can be seen from the results of only controlling the indirect effect of the DE on MLC. As its geographic and economic distance matrices are inverse indicators, the indirect effect coefficient of the DE is negative. Therefore, the development of the DE can reduce the geographic specificity of MLC, reduce the frequency of transactions between coastal areas, reduce the uncertainty in coastal areas’ response to the unbalanced development pattern, and create a positive spatial spillover effect on MLC. Through the development of the DE, the spatial and coastal provincial system can be moved from unbalanced to integrated, which will have a positive incentive for the MLC of the surrounding marines. Notably, during the operation of this system, the marine low-carbon development will not be spilled over by the agglomeration of adjacent industries. However, the development of the DE can allow coastal provinces to absorb production factors from adjacent coastal locations to increase the marine low-carbon level.
Robustness testsPrevious empirical results have confirmed that the research rationale of this study is, to some extent, valid. The robustness test is as follows. The first step is to re-estimate the model incorporating Internet coverage as an explanatory variable (1). The second step is to reduce the effect of endogeneity on the outcomes. The systematic generalized method of moments (GMM) approach will relieve the endogeneity problem in this research. The systematic GMM concept utilizes the level and different values of the endogenous explanatory variable as the instrumental variable to relieve the endogeneity problem without selecting other instrumental variables. The third step is to swap out the spatial weight matrix. As various weight matrices might have different impacts on regression findings, this article replaces the 0–1 matrix with a geographic matrix to analyze the spatial influence of the DE on carbon emissions.
Model (1) of Table 9 displays the study findings on the Internet coverage rate in the marine economy. The Internet coverage regression coefficient is found to be positive at the 1% significance level, and the U-shaped association between the DE and MLC is still evident. The estimation results are shown in Model (2) of Table 9 from the systematic GMM method. AR(2) adjoin probability and Sargan test results are more significant than 0.05, indicating no second-order linear relationship between the variables in the residual elements. The systematic GMM's moment condition is that there should be no risk of over-identifying instrumental variables, and the system GMM estimate findings are acceptable. After accounting for endogeneity, the value of the DE coefficient remains significant at 5%. The systematic GMM method estimations revealed that the presence of the DE on the MLC contribution to the marine environment is robust. Model (3) of Table 9 displays the regression analysis after changing the space matrix. The coincidence and significance of each explanatory variable are consistent with the preceding overall, and the importance is more significant, supporting the robustness of the conclusions in this research.
Robustness test.
Note: Robust standard errors are in brackets; ***, **, and * indicate significance at the 1%, 5%, and 10% levels, respectively.
According to empirical studies, the growth of the DE has a beneficial influence on the MLCs. The technological advancements carried about by the DE will assist firms in resetting manufacturing equipment in the early phases of economic development, raising production by increasing resource extraction and energy consumption, and therefore growing carbon emissions (Jorge et al., 2022). When a greater degree of development is reached, the company output is constant, and digital technology and scale reduce the cost of pollution management. This case results in a decrease in carbon dioxide emissions, demonstrating the DE's nonlinear impact on carbon emissions. Through the optimization of IST and the enhancement of IE, the DE has a positive effect on the MLC.
The beneficial influence of the DE on the MLC is mainly accomplished by enhancing the degree of IE and IST. The practical effect of the DE on the efficiency with which carbon emissions are reduced is achieved primarily by improving the degree of IE and optimizing the industry's structure. The development of platform economy, sharing economy, and other formats in the DE is conducive to strengthening market competition, accelerating the logical flow and optimal combination of resource elements, and improving the coupling between industrial structure and resource element ratio (Yao et al., 2022). Simultaneously, the fast growth of Internet trading platforms has overcome the temporal and spatial restrictions of conventional trade, expedited the cross-regional flow of production components, and broadened the scope of resource allocation. As a result, optimizing industrial structures can effectively lower environmental pollution emissions. From another aspect, the development of the DE has gradually improved the digital infrastructure, and the widespread application of digital technologies, such as search engines and cloud storage, has made it easier for businesses, universities, and other innovative organizations to obtain and manage information. This case reduces the cost of searching and working on external knowledge and accelerates the accumulation of knowledge based on predecessors, thereby improving IE. In addition, the DE breaks down the barriers to information flow. This case enables a more timely exchange of knowledge among innovative entities and optimizes the management efficiency of R&D teams. In addition, this phenomenon enables innovative entities to fully capture market feedback on products and services and understand the market's response to new technologies. Therefore, product R&D needs and promotes the improvement of IE.
ConclusionsFirst, this article theoretically examines how DE influences carbon emission efficiency. Second, the DEA-Malmquist index and the entropy weight method are used to evaluate marine low-carbon efficiency and DE development. This analysis utilized a mediation effect model and a spatial spillover model to conduct empirical research on the effects and strategy of the DE on the efficiency of marine carbon emissions. The results of the study indicate the following: First, the impact of the DE on the MLC has a U-shaped nonlinear relationship that first inhibits and then promotes, and regional heterogeneity exists. Second, the DE has an indirect impact on MLC through industrial structure and IE. Third, the regression indicates that the spatial Durbin model shows that the U-shaped nonlinear effect of the DE on carbon emission efficiency continues to remain after taking into spatial account factors. Moreover, when compared with the DE's spillover effect, the direct impact of the DE on local carbon emissions is more prominent. Based on the research findings, the following suggestions are made: Increase regional carbon emission reduction exchange and collaboration to continue. The efficiency of carbon emissions in various locations is connected. Therefore, a sound exchange and cooperation mechanism between regions and project exchanges and cooperation should be strengthened. Second, the structural adjustment of the marine industry needs to be actively promoted. On the one hand, traditional businesses, such as maritime, marine fisheries, coastal tourism, marine oil and gas, and marine chemical industries, continue to dominate the growth of China's marine economy, according to the “2018 China Marine Economy Statistical Bulletin.” As a result, to increase the marine's low-carbon efficiency, the traditional marine industry must undergo a low-carbon technological revolution.
On the other hand, vigorously developing the marine service and technology industries is necessary. According to a statistical analysis of China's maritime economy, China's present essential marine developing industries have an annual yearly growth rate of more than 20%. However, their small scale is less than 2% of the gross marine economy. Moreover, the proportion of the tertiary industry should be improved, and marine service industries, such as coastal tourism, recreational fisheries, and marine medicine, need to be promoted. Third, the improvement of IE should be focused on. The research revealed that IE had a partial mediation effect on low-carbon efficiency in the DE. More attention should be devoted to IE growth while fostering low-carbon marine efficiency development. To ensure the high quality of innovation output by colleges and universities, it is vital to plan scientifically and rationally distribute colleges and universities, ensure that innovation input is active and moderate, and limit input and output loss. Moreover, new efficiency transformation mechanisms must be developed, and communication and collaboration with businesses should be improved.
Funding statementThis research was funded by Guangdong Province Philosophy and Social Sciences Planning Project (Grant Number: GD20XYJ22), Guangdong Philosophy and Social Sciences Planning Youth Project (Grant Number:GD22YGL19), Planning project of Guangdong Department of Education (Grant Number: 2019WQNCX040), and Humanities and Social Sciences Project of Guangdong Ocean University (Grant Number: C20116).
Author contributionsConceptualization, Wei Yao and Wenxiu Li; methodology, Weikun Zhang; software, Wei Yao and Weikun Zhang; validation, Wei Yao, Weikun Zhang, and. Wenxiu Li formal analysis, Wei Yao; investigation, Weikun Zhang; resources, Wei Yao and Wenxiu Li; data curation, Wei Yao; writing—original draft preparation,Wei Yao and Weikun Zhang; writing—review and editing, Weikun Zhang and Wenxiu Li; visualization, Wei Yao; supervision, Wenxiu Li; project administration, Wenxiu Li; funding acquisition, Wenxiu Li.
All authors have read and agreed to the published version of the manuscript.
Data availability statementThe data used to support the findings of this study are included within the article.
CRediT authorship contribution statementWei Yao: Conceptualization, Software, Validation, Formal analysis, Resources, Data curation, Writing – original draft, Visualization. Weikun Zhang: Methodology, Software, Validation, Investigation, Writing – original draft, Writing – review & editing. Wenxiu Li: Conceptualization, Validation, Resources, Writing – review & editing, Supervision, Project administration, Funding acquisition.